The meeting is called ESPP and it is an INFN effort to gather input from its community on the next European strategy update for particle physics. On Tuesday I will give there a short talk on AI for detector design, to stimulate the ensuing scheduled discussion. What I would like to say and what I will say are this time two slightly different things, because in the little time I am given the risk of being misunderstood - or worse, to offend the community of detector builders - is too high.
In this safe spot, far from the sight of those sensitive souls, I can however try to explain what I would really like to say tomorrow. I reckon that this apotropaic ritual can somehow help me take the unnecessary controversial pitch off my speech, and make it more acceptable and clear.
The gist of what I would like to say, in my typical outspoken fashion, is the following: move over already. In case you have been on a coma for the past few years (as M.P. aptly put it during a conversation at EUCAIF last week), you might not have noticed that an AI revolution is impending on us. This demands swift action, such that we manage to adapt to the rapidly evolving situation everything we do which has an impact on society. Otherwise, the risk we run is to produce outcomes that will end up being misaligned, suboptimal, or just wrong. So either embrace it or leave it.
I know, it is a bit hard to follow the above train of thoughts, so let us unpack it a bit.
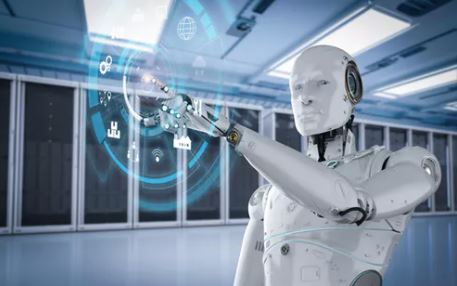
Chim/Shutterstock
1. Through half a century of successful practice, particle physicists have become confident at conceiving, building, and operating enormously complex experiments that leverage state-of-the-art technologies (and sometimes by envisioning entirely novel ones).
2. Due to our greed for digging deeper in the mysteries of subnuclear matter, we have progressively pushed the scale of those endeavours, so that the turnaround time from blueprint to exploitation of the instruments has consequently moved from the few years to the tens of years range - making the periodic update of our strategy plans, indeed, a meaningful thing to entertain ourselves with.
3. Even in a stationary state, designing an instrument that will operate in 20 years requires vision: how will the political, economic, and scientific situation evolve from now to twenty years down the line? Physicists have grown used to extrapolate those factors, with mixed successes and at least one big failure, the SSC - which got canceled by an adverse US Congress vote in September 1993. Ok, that was almost entirely not our fault, yet it is an episode that shows how thin the ice is, even in normal times.
4. But we are not in normal times. In 20 years, the software that will make sense of the data our instruments produce will be a far cry from what we have in our hands today. That software will be capable of extracting information about particle interactions with matter that we today do not yet care to enable or preserve (An example? we disallow nuclear interactions while we track charged particles, by making our trackers as lightweight as we can. A good idea, but the abrupt transition to high density of the calorimeters down the line needs a rethink in a situation where AI can make sense of particle ID by exploiting those nasty nuclear hits). What we are risking, therefore, is to design instruments today that will be misaligned with the capabilities of future information extraction and processing tomorrow.
So, how should we approach the problem? To me the answer is a no-brainer: by embracing the evolving new AI technology, and trying to surf that huge wave coming at us. But are we equipped to do that? Alas, yes but no - many in the high-energy-physics community have understood the opportunities and the dangers of the AI maelstrom, but our detector building experts are typically not very keen on toying with emerging software technologies.
In the end, those who will call the shots on what and how to build, for experiments that will operate in 20 years from now, are colleagues who are maybe a decade older than me. Close to retirement, they want to have an impact, and they will. But if they neglect the incoming wave, they will shape a future of particle detectors that risks to be suboptimal.
I already explained here some time ago that I suffered a strong cognitive dissonance five years ago, when I first heard my colleague Franco Bedeschi describe the design he had envisioned for a future detector operating at a higher-energy electron-positron collider, during a board meeting of INFN "Commissione 1" in Catania. Besides being a high-esteemed colleague and friend, Franco is one of our maximum experts of particle detectors, but he will be retired when such a machine is eventually built. In Catania he was not paying any attention to the elephant in the room - AI - when he put his ideas together.
So that is what I mean when I cry, "Move over already". That is a figurative, though a bit abrasive, form of expressing it: I do not really want Franco or his contemporaries to move over. Rather, I would like them to pay as much attention as they can, and then some, to ensure that the design of future detectors is actually a co-design of detectors and software.
Wait, what? Didn't I spent the past umpteen lines of text pounding on the concept of us being unable to predict the future of our software capabilities? Why then do I now want to insert present software in the pipeline?
I did not say "present", doh. In fact, I have been arguing that it is a very good ansatz to assume perfect reconstruction performance of our software tools when we create a pipeline modeling our detector parameters, the particle interactions, and the information extraction from the detector readouts. Such a pipeline can then be used to optimize the detector parameters in an end-to-end fashion, to discover what construction choices would potentially allow for higher performance (as measured by the precision of final results, such as "highest discovery reach for new physics" if we are building a detector for a future high-energy collider, e.g.). The process of degrading perfect reconstruction to state-of-the art can then be inserted in the modeling chain, to appraise what modification those changes suggest to the design, and to understand how robust the identified optimal choices are.
The above may seem a rather contrived procedure. The problem is that due to the complexity of our instruments, we cannot run our high-fidelity simulations to explore every point in the high-dimensional space of design choices. We need to rather allow some automated scanning procedure - powered by gradient descent, deep learning functionalities - to do the job. This requires easing up on the accuracy of the modeling, in favor of more powerful and thorough investigation of the configuration space.
To summarize: co-design of hardware and software, and end-to-end optimization of the resulting systems, should be the way we take in designing our future experiments. This is already being done in industrial applications, where there are more resources for R&D in software engineering. In our field, however, it is not the norm, mainly because it is damn hard to do it! But we need to start doing it systematically, instead. For sure, as a community we are not one that is easily scared by grand challenges!
My own little contribution to the above call to arms is having founded the MODE collaboration, and tackling a few not-too-hard design optimization tasks. And last week, at EUCAIF we have started the activities of one of five working groups, centered on precisely the topic of co-design for future experiments. I am leading that effort with Pietro Vischia, and we hope to reach out to our community and make it aware of the potential AI bears for fundamental science.
I do hope this message gets through, and after writing the above blurb I think I can more clearly make the point in my talk tomorrow.
Comments