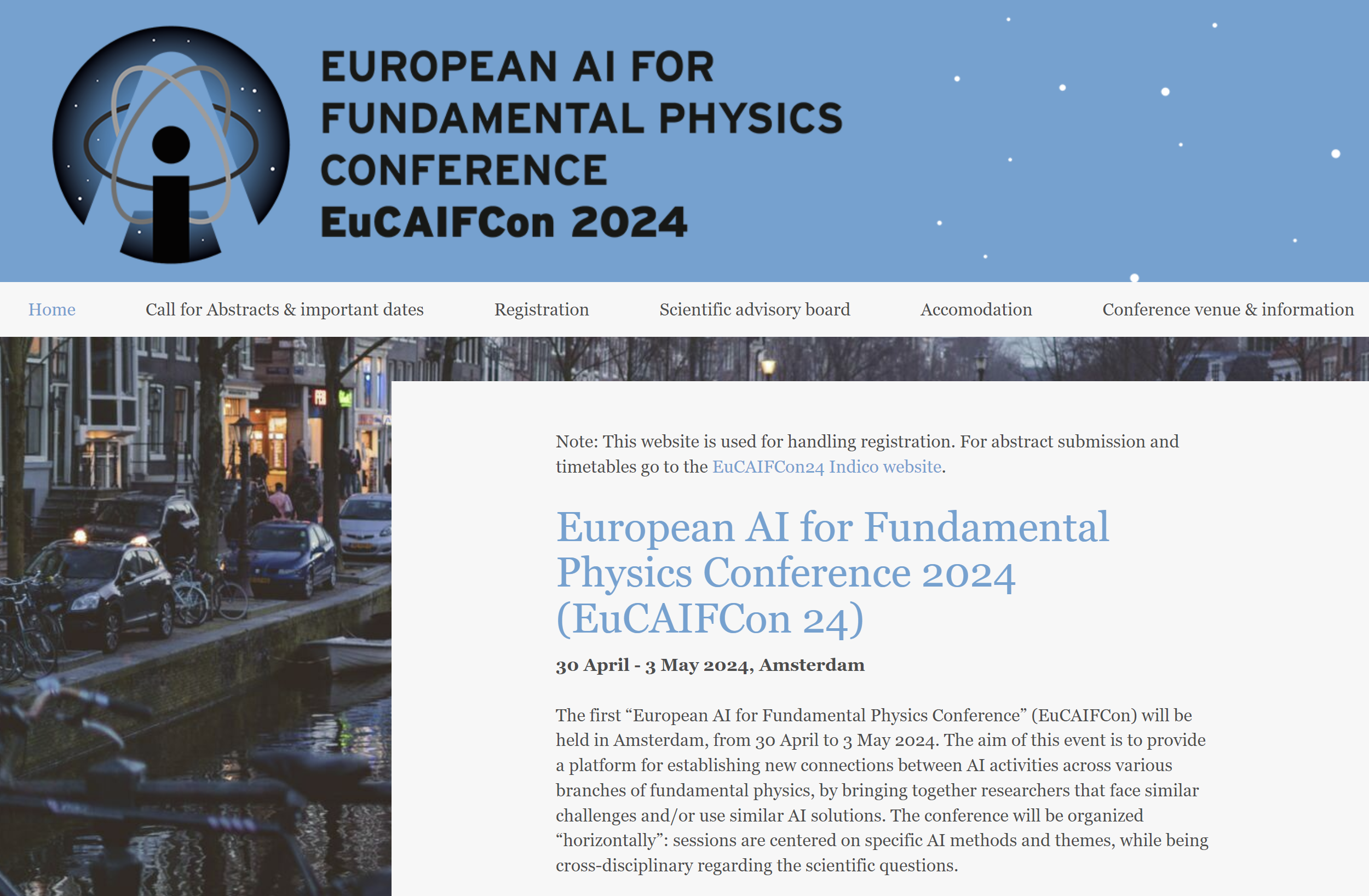
Our group, led by Sascha Caron and Christoph Weniger from the University of Amsterdam, sees the participation of several leading scientists who operate in the fields of theoretical physics, experimental physics at particle colliders, gravitational wave detectors, nuclear and neutrino physics, and astroparticle physics. We organized the EUCAIF conference as a launch platform for a more coherent effort to organize the application of AI methods to reach our scientific research goals, as we have observed that a qualitative step forward is possible by exploiting existing synergies in problem settings that are only apparently different.
Physicists have a long tradition in being problem solvers and factotums. By asking ourselves hard questions about the structure of matter and the working of the universe, we use to set the bar extremely high to ourselves. This forces us to exploit state of the art technologies, when they exists, or to invent ones anew otherwise: such was the story around the construction of high-vacuum glass tubes in the nineteenth century (which enabled discoveries of x-rays, of the electron, and the study of the photoelectric effect), or around the development of radiation-tolerant silicon detectors (which were instrumental e.g. in the discovery of the top quark).
Similarly, roughly until the 1980ies the complex computing demands of particle colliders demanded physicists to become software specialists and develop high computer programming skills, and to also double up as custom computing hardware developers. After that, however, it started to become clear that our time was better invested elsewhere, and that off-the-shelf computers were the cheapest and most practical way to instrument our experiments. But we have never stopped writing (ugly) code!
Today, a similar trend is happening with the new technology in town - artificial intelligence. We have been using machine learning techniques in the past 40 years for our data analysis tasks, and we still do (and enjoy it a lot), but we also do understand that the huge profits of new AI developments are having a shearing effect between what we can do on our own and what is becoming possible if you have the huge resources of Google, OpenAI, and other big players.
To bridge this gap, and secure an access for fundamental science to the state of the art in AI technology, some of us have been trying to build links with computer scientists, getting them interested in our specific problems, and creating lasting collaborative efforts. For our problems are kind of special - they typically cannot be solved by just downloading the next version of LLama or some other large language model. What we need is expert vision on what are the best ways to use the new available technologies, and how to ensure that our research remains capable of exploiting it in the future.
My little contribution to the above was the founding of the MODE collaboration, four years ago. With a few colleagues in a handful of institutes in Europe and the US we started working at how to use deep learning tools - powered by differentiable programming languages - to create full models of our experiments. The idea is that it is today possible to design a particle detector in a way that it is not just good, but the best possible detector you can build for a specific task. To do it, you need to consider everything - from the physics processes that produce the information you want to harvest, to the geometry and working of your detection instruments, to the software that extracts the information and produces the final results.
MODE has grown to include over 40 members from about 30 institutes around three continents, and we have a long list of projects we are developing, where we seek to optimize end-to-end the design of instruments for fundamental science - from muon tomography, to hadron calorimeters, to ground arrays for gamma-ray detection, to future detectors for the muon collider. We are also exploiting the potential of entirely new computing paradigms, such as neuromorphic computing.
But the effort of MODE is only targeting a narrow area of the galaxy of problems that researchers in fundamental science want to solve with artificial intelligence. By calling on all young bright physicists around who understand the importance of AI for science, EUCAIF is our opportunity to organize our effort toward retaining access to cutting edge AI methods. Of course, this can only be done if we engage AI researchers in the endeavour, and it is a challenging part of our plan.
At EUCAIF, I will be co-chairing one of the four working groups that we have defined to move forward, and of which the conference will be the initial kick-off act. The working group is titled "AI-assisted co-design of future ground- and space-based detectors", and the three defining questions around which it will be organized are the following:
1) "Identify existing design paradigms for particle and astroparticle physics instruments which have become obsolete in the AI era, and assemble software strategies and research paths to overtake them"
2) "Support the development of simulation tools that constitute enablers of co-design approaches to holistic optimization for detector use cases in HEP, astro-HEP, nuclear and neutrino physics."
3) "Understand physical limits of information generated by particle interactions in granular calorimeters and conditions for its lossless extraction, as a preliminary step toward the AI-assisted hybridization of calorimeters and tracking detectors into optimized variable-density systems".As you see, we will have our hands full in getting this done in the coming years! I look forward to the initial discussion we will have next Tuesday afternoon on how to get started...
Comments