A group of researchers, led by Martha Bulyk (Harvard) and Tim Hughes (Toronto) have been working towards a near-comprehensive index of DNA sequence specificities for DNA-binding proteins. The goal is to systematically explore DNA 'sequence-space' with each DNA binding protein, and identify those DNA sequences that are bound by a given protein. This is done using Protein Binding Microarrays - you put DNA on a chip, and wash purified protein over it. Using fluorescent labels, you can identify which snippets of DNA the proteins stick to.
The group has a paper out in this week's issue of Science, reporting their results for mouse regulatory proteins. So what lessons do we learn about gene regulation?
1) Many regulatory proteins recognize more than one DNA sequence motif. About half of the transcription factor proteins tested bound to two distinct DNA motifs. This is a little weird, but not unknown - it makes things more complicated. What makes things really weird is:
2) Individual DNA bases in the motif are not independent in some cases. The authors explain what that means:
For example, estrogen related receptor alpha has a strong preference for binding either CAAGGTCA or AGGGGTCA, but not CAGGGTCA or CGGGGTCA.
This is heresy! (Did you miss it? Read the quote again.) Well, maybe it's not heresy (things are the way they are; who are we to argue?), but this is a real pain. Here's why: transcription factor binding specificities are actually degenerate (meaning that there is always a little variation in the DNA sequence that can be bound) - maybe a protein can bind CAACGG and CAGCGG, and a few other variants. This specificity is usually represented graphically by a weblogo:
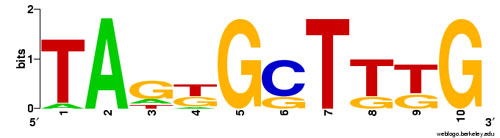
The weblogo is just a visual way of showing an underlying probabilistic model. To build these models, you collect dozens of known DNA binding sites, align those sites with each other like this (each row is an actual sequence of DNA from some gene's promoter):
TAGTGGTTGG
TAAGGCTTGG
TAGGGCTTTG
TAGGGCTTGG
TAAGGCTTGG
TATTGGTTTG
TAGTGCTGTG
TAGGGGTGTG
TATTGCTTTG
AAGTGCTTTG
TAGTGCTTTG
TAGAGCTGTG
And then you build the mathematical model, from which you can predict how likely it is that your transcription factor will bind a given stretch of DNA that resembles known binding sites. To build that model, you assume independence of each DNA base in the site: if you have a 'G' at one position, it doesn't matter whether you have an 'A' two positions away; that 'G' contributes a given amount of energy to the binding affinity no matter what the context.
Using models like this, you can scan through a genome and predict where all of the transcription factor binding sites are, and thus predict which transcription factors regulate which genes - thereby identifying the genetic regulatory networks that control cellular processes.
But if our assumption of position interdependence is wrong, we're screwed, because it's much hard to build good models, given the data we have, without that assumption. (As a rule, building non-additive models of anything in biology is tricky.)
Well, maybe we're not completely hosed: in the Bulyk paper, it looks like about 18% of the transcription factors they examined showed position interdependence (i.e., non-additive effects) - so for most regulatory proteins, the assumption of independence should be just fine. And in fact we have had a lot of success with the current models, so clearly the assumptions that go into them work in many cases.
Nature is what it is, and in molecular biology, there are exceptions to just about any general rule you can come up with - so these results aren't too surprising. But they serve as a warning: we can't be satisfied with a single type of model to understand transcription factor-DNA binding. In biology, there is never one solution that works for everything.

Comments