But if you ask a child or someone from a culture not trained in maths, the answer could be different; perhaps 3. It isn't that they don't know how to count, it's because it's actually more natural for humans to think logarithmically instead of linearly, say researchers. Neural circuits seem to bear out that hypothesis; in psychological experiments, multiplying the intensity of some sensory stimuli causes a linear increase in perceived intensity.
30 is 1, and 32 is 9, so logarithmically, the number halfway between them is 31 or 3 - who knew people with no training were so smart? We may not know it, but our brains are, and researchers from MIT's Signal Transformation and Information Representation group recently used information theory to demonstrate that, given certain assumptions about the natural environment and the way neural systems work, representing information logarithmically rather than linearly reduces the risk of error. Why are signal-processing people, who usually work with optical imaging or magnetic resonance imaging, publishing a paper in a psychology journal?
"Although this problem seems very removed from what we do naturally, it's actually not the case," says graduate student John Sun. "We do a lot of media compression, and media compression, for the most part, is very well-motivated by psychophysical experiments. So when they came up with MP3 compression, when they came up with JPEG, they used a lot of these perceptual things: What do you perceive well, what don't you perceive well?"
One of the researchers' assumptions is that if you were designing a nervous system for humans living in the ancestral environment, with the aim that it accurately represent the world around them, the right type of error to minimize would be relative error, not absolute error. After all, being off by four matters much more if the question is whether there are one or five hungry lions in the tall grass around you than if the question is whether there are 96 or 100 antelope in the herd you've just spotted.
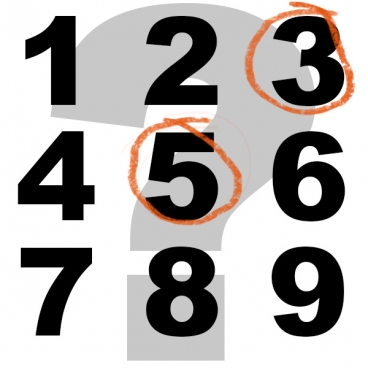
Graphic: Christine Daniloff
The STIR researchers demonstrated that if you're trying to minimize relative error, using a logarithmic scale is the best approach under two different conditions: One is if you're trying to store your representations of the outside world in memory; the other is if sensory stimuli in the outside world happen to fall into particular statistical patterns.
If you're trying to store data in memory, a logarithmic scale is optimal if there's any chance of error in either storage or retrieval, or if you need to compress the data so that it takes up less space. The researchers believe that one of these conditions probably pertains — there's evidence in the psychological literature for both — but they're not committed to either. They do feel, however, that the pressures of memory storage probably explain the natural human instinct to represent numbers logarithmically.
In their paper, the researchers also looked at the statistical patterns that describe volume fluctuations in human speech. As it turns out, those fluctuations are well approximated by a normal distribution — a bell curve — but only if they're represented logarithmically. Under such circumstances, the researchers show, logarithmic representation again minimizes the relative error.
The STIR researchers' information theorymodel also fits the empirical psychological data in other ways. One is that it predicts the point at which human sensory discrimination will break down. With sound volume, for instance, experimental subjects can make very fine distinctions within a range of values, "but experimentally, when we get to the edges, there are breakdowns," Sun says.
Similarly, the model does a better job than its predecessors of describing brain plasticity. It provides a framework in which a straightforward application of Bayes' theorem, the cornerstone of much modern statistical analysis, accurately predicts the extent to which predilections hard-wired into the human nervous system can be revised in light of experience.
Citation: John Z. Sun, Grace I. Wang, Vivek K Goyal, Lav R. Varshney, 'A framework for Bayesian optimality of psychophysical laws', Journal of Mathematical Psychology In Press, Corrected Proof DOI:10.1016/j.jmp.2012.08.002
Comments