Instead, we got a coronavirus from Wuhan, China, and a COVID-19 disease that isn't stopping any time soon. But science marches on, and we also have machine learning helping to grow artificial organs.
The human retina has a very limited capacity for regeneration. Any progressive loss of neurons, such as in glaucoma, inevitably leads to complete loss of vision. There is nothing a physician can recommend to halt it. But science is on the case. In a recent study, a neural network capable of recognizing retinal tissues during the process of differentiation does so without the need to modify cells, making it ideal for growing retinal tissue for developing cell replacement therapies to treat blindness and conducting research into new drugs.
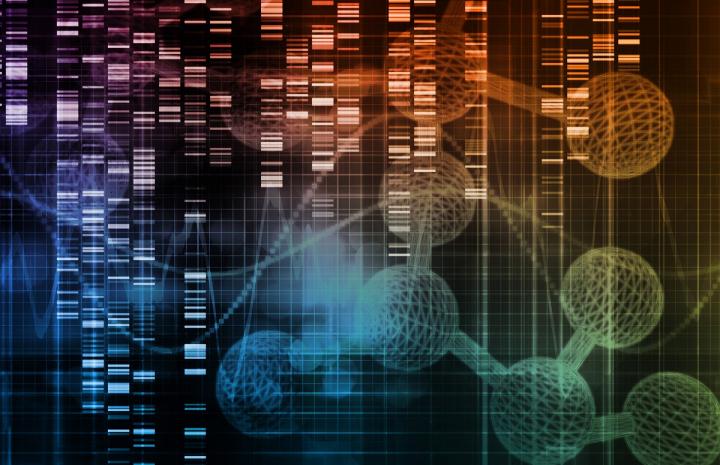
Credit: MIPT Press Office
That's more complex than it reads at first. In multicellular organisms, the cells making up different organs and tissues start the same, but then acquire distinct functions and properties in the course of development. Stem cells have the potential to become any kind of cell, they undergo differentiation by producing proteins specific to certain tissues and organs.
The most advanced technique for replicating tissue differentiation in vitro relies on 3-D cell aggregates called organoids. Since organoid-based differentiation closely mimics natural processes, the resulting tissue is very similar to the one in an actual biological organ. Some of the stages in cell differentiation toward retina have a stochastic (random) nature, leading to considerable variations in the number of cells with a particular function even between artificial organs in the same batch. The discrepancy is even greater when different cell lines are involved. As a result, it is necessary to have a means of determining which cells have already differentiated at a given point in time. Otherwise, experiments will not be truly replicable, making clinical applications less reliable, too.
To spot differentiated cells, tissue engineers use fluorescent proteins. By inserting the gene responsible for the production of such a protein into the DNA of cells, researchers ensure that it is synthesized and produces a signal once a certain stage in cell development has been reached. While this technique is highly sensitive, specific, and convenient for quantitative assessments, it is not suitable for cells intended for transplantation or hereditary disease modeling.
To address that pitfall, the authors of the recent study proposed an alternative approach based on tissue structure. No reliable and objective criteria for predicting the quality of differentiated cells have been formulated so far. The researchers proposed that the best retinal tissues, those most suitable for transplantation, drug screening, or disease modeling, should be selected using neural networks and artificial intelligence.
The team trained a neural network - a computer algorithm that mimics the way neurons work in the human brain - to identify the tissues in a developing retina based on photographs made by a conventional light microscope. The researchers first had a number of experts identify the differentiated cells in 1,200 images via an accurate technique that involves the use of a fluorescent reporter. The neural network was trained on 750 images, with another 150 used for validation and 250 for testing predictions. At this last stage, the machine was able to spot differentiated cells with an 84% accuracy, compared with 67% achieved by humans.
"Our findings indicate that the current criteria used for early-stage retinal tissue selection may be subjective. They depend on the expert making the decision. However, we hypothesized that the tissue morphology, its structure, contains clues that enable predicting retinal differentiation, even at very early stages. And unlike a human, the computer program can extract that information!" states Evgenii Kegeles of the Moscow Institute of Physics and Technology. "This approach does not require images of a very high quality, fluorescent reporters, or dyes, making it relatively easy to implement. It takes us one step closer to developing cellular therapies for the retinal diseases such as glaucoma and macular degeneration, which today invariably lead to blindness. Besides that, the approach can be transferred not just to other cell lines, but also to other human artificial organs."
Comments