- Sean Benson, Netherlands Cancer Institute
- Thomas Breuel, Nvidia
- Hao Chen, Hong Kong University of Science and Technology
- Janlin Chen, University of Missouri
- Nadya Chernyavskaya, CERN
- Efstratios Gavves, University of Amsterdam
- Quanquan Gu, University of California Los Angeles
- Jiawei Han, University of Illinois Urbana-Champaign
- Awni Hannun, Zoom
- Tin Kam Ho, IBM Thomas J. Watson Research Center
- Timothy Hospedales, University of Edimburgh
- Shih-Chieh Hsu, University of Washington
- Tatiana Likhomanenko, Apple
- Othmane Rifki, Spectrum Labs
- Mayank Vatsa, Indian Institute of Technology Jodhpur
- Yao Wang, New York University
- Zichen Wang, Amazon web services
- Alper Yilmaz, Ohio State University
In addition to the lectures offered by the above academics and industry specialists, the organizers invited two keynote speakers to discuss some cutting-edge developments: Elaine O. Nsoesie from Boston University, and yours truly. So I am spending a few days in proximity of the arctic polar circle, and this afternoon I will deliver my keynote lecture. What will I talk about?
The title of my talk is easy to guess: "Deep-Learning-Optimized Design of Experiments: Challenges and Opportunities". Below I will give a few highlights from the material, and I hope I will later be able to link to a recorded version to the three readers who are interested (to those three of you: if I fail to link it, please bug me about it by email).
My lecture
Since I will be talking to students with quite different backgrounds, who come to learn about deep learning for many different disciplines, I will have to introduce the topic of particle physics. I have a few slides of introduction where I explain what the standard model is, what we do at the LHC, how we detect particles, and how we make sense of them. The slide below summarizes this latter bit. It is meant to be animated (one bit at a time) but here you get to see it all in one swoop:
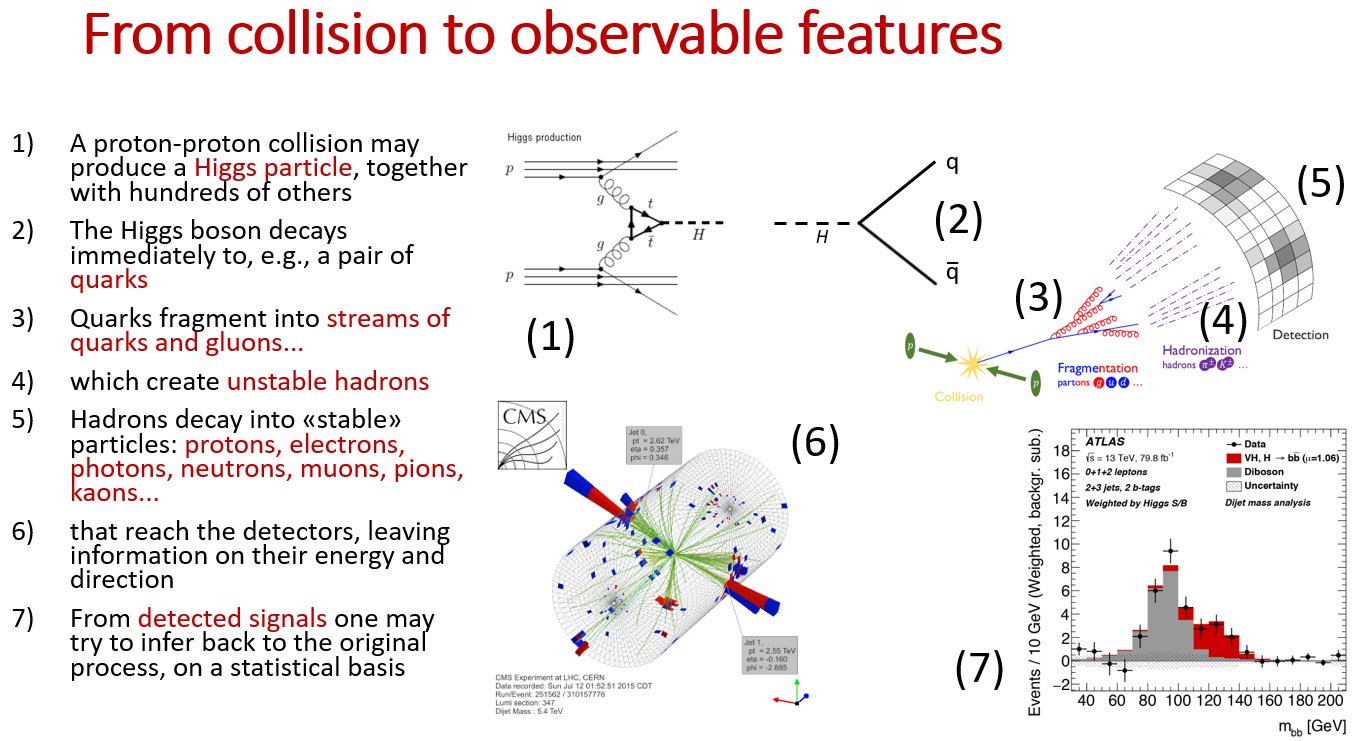
Then I discuss how deep learning is presently used in particle physics, and how the topic I want to discuss moves the bar a bit higher:
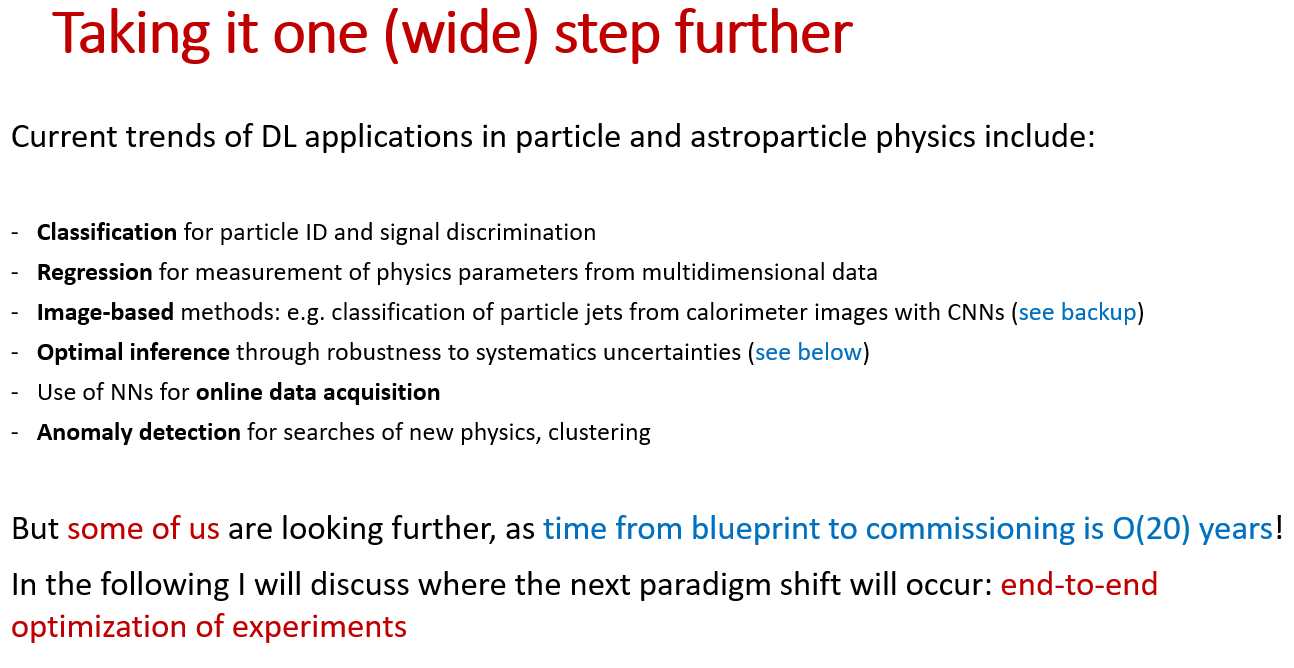
I discuss the needs of this new area of research by explaining that building performant detectors is very complex, and to do it we rely on experience and some long standing paradigms that have helped us in the past 50 years or so, but which do not aim for optimality - rather, they seek robustness and redundancy. So moving away from those paradigms, we can imagine to gain a lot by continuous scanning of the very high-dimensional parameter space of possible design choices. One important part of it is defining all aspects of the problem with differentiable functions. This may look naive, but it is a very good exercise to force yourself to declare what your objectives are - something that if left hanging will not produce the desirable results.
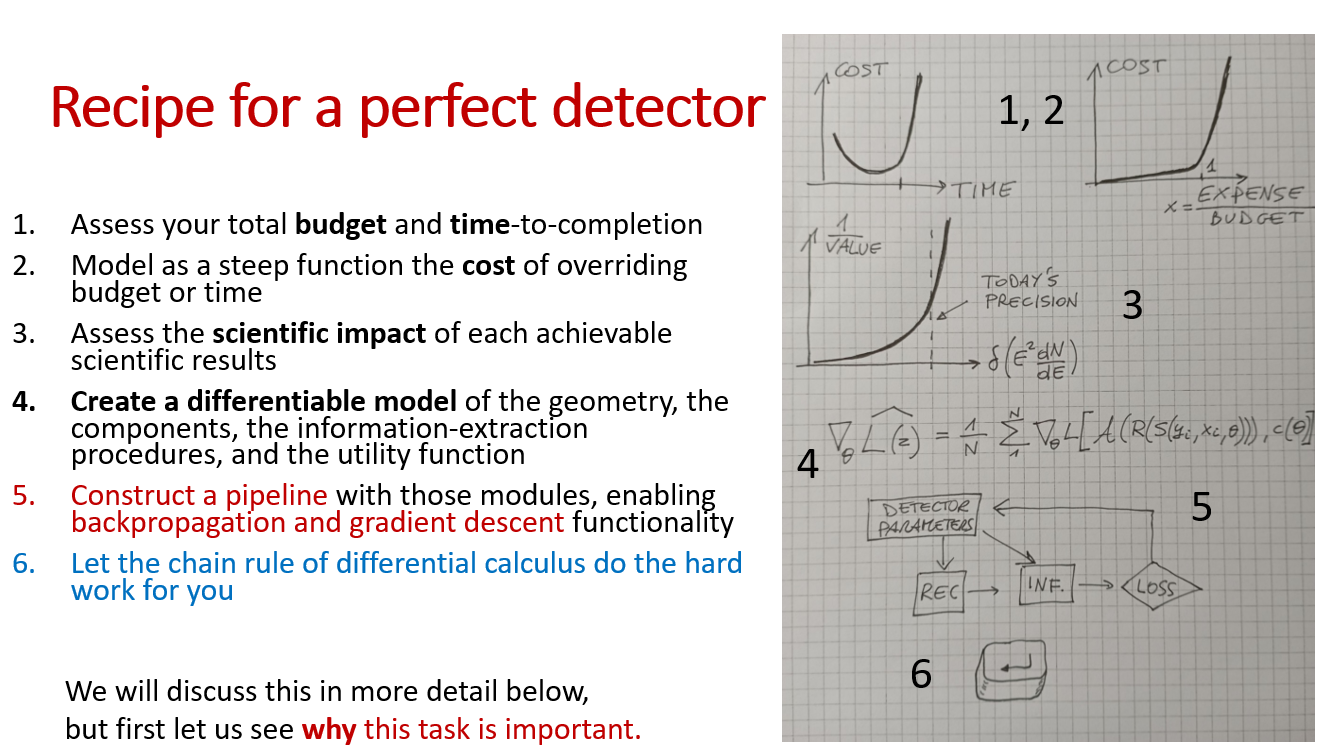
I then deviate to discuss one optimization task I took on by myself two years ago, when I could prove that an experiment under design could be designed better - to do that I had to create a fast simulation of the whole experiment and physics, and then study the various choices. I proved that the relevant figure of merit could be improved by a factor of two without increase in the cost, and now the collaboration is adopting many of my suggestions.
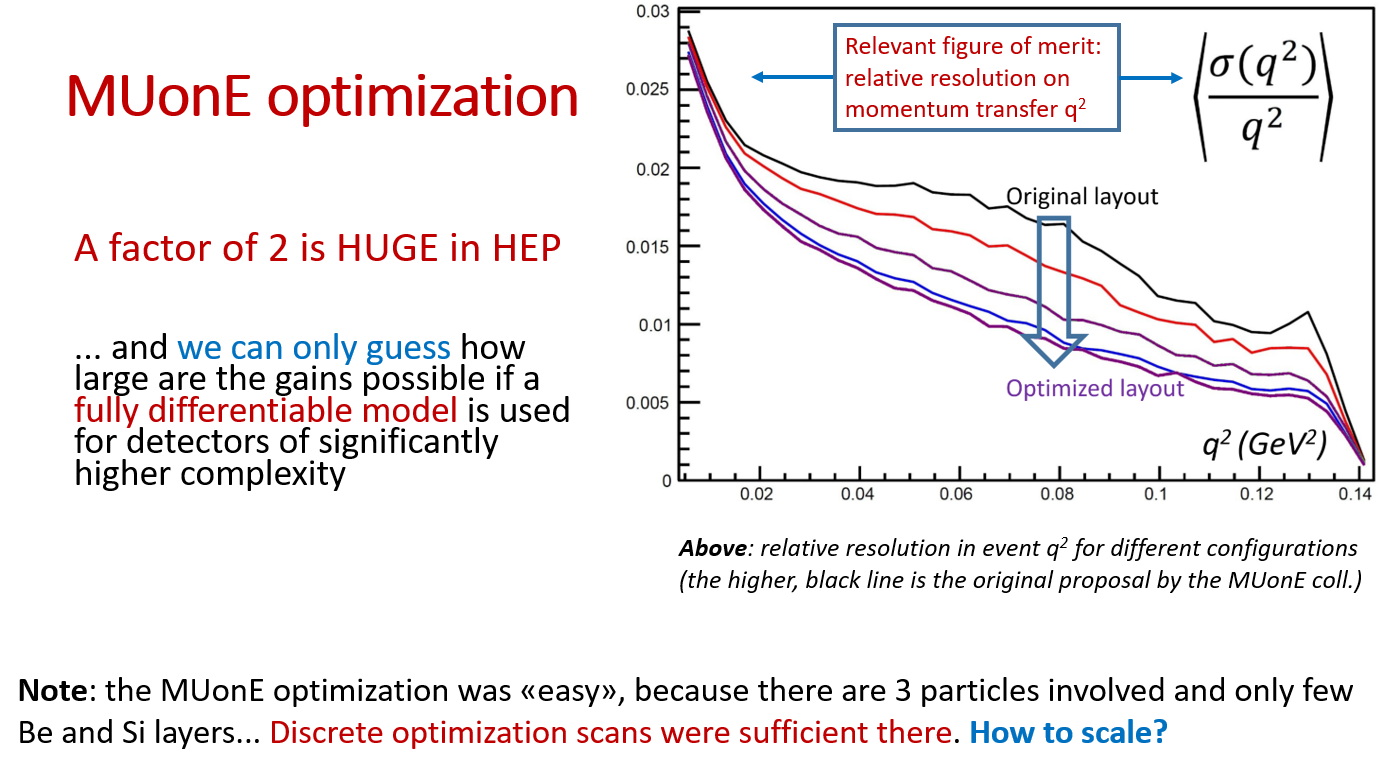
I then discuss in some detail how the problem can be formalized in general, with some maths. I will spare that to you, and just tease you with one of the slides where I discuss how the optimality can be sought in the configuration space.
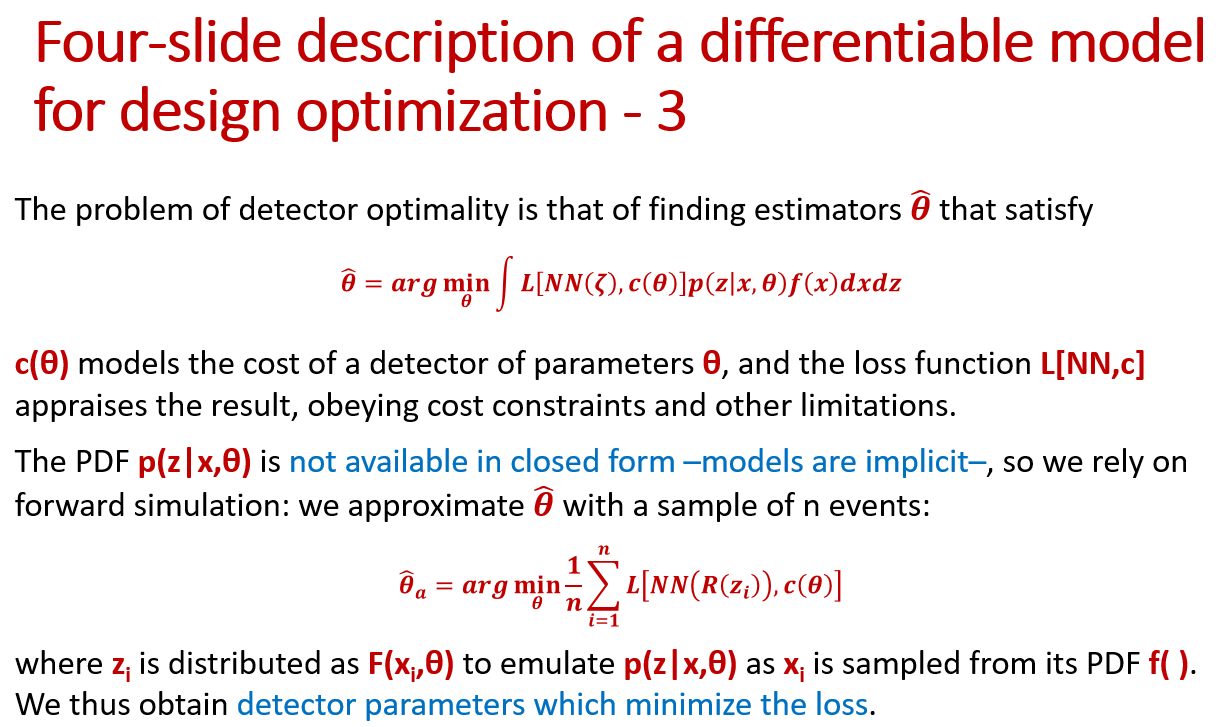
I then show an example of how the above recipe can be put to work. This is great work by some members of MODE who work for the LHCb experiment (Ratnikov, Derkach, Boldyrev, Ustyuzhanin). They created a generative adversarial network emulation of EM showers in the calorimeter, in order to insert that in a differentiable pipeline optimizing the geometry of the apparatus.
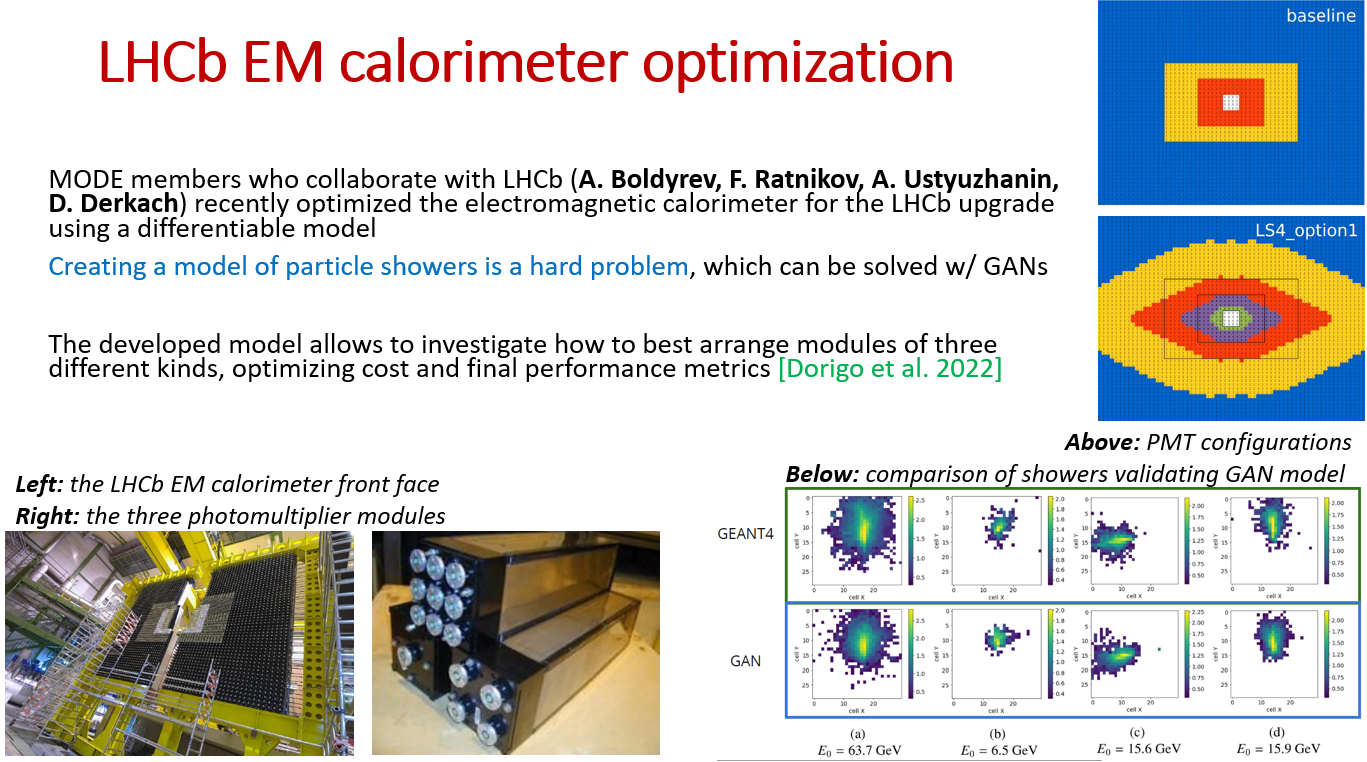
Then I discuss a project I initiated with a few colleagues from Padova, Louvain-la-Neuve, and elsewhere. The topic is the inference of the density map of unknown volumes by scattering tomography. You track a muon going through the volume, and understand how dense was the material it encountered by the amount of scattering it withstood. Optimizing the "simple" detectors that do this is less complex than doing it for a collider, but the challenges are similar, and solving easy use cases allows us to focus on the real hurdles, gaining confidence and building a library of solutions.
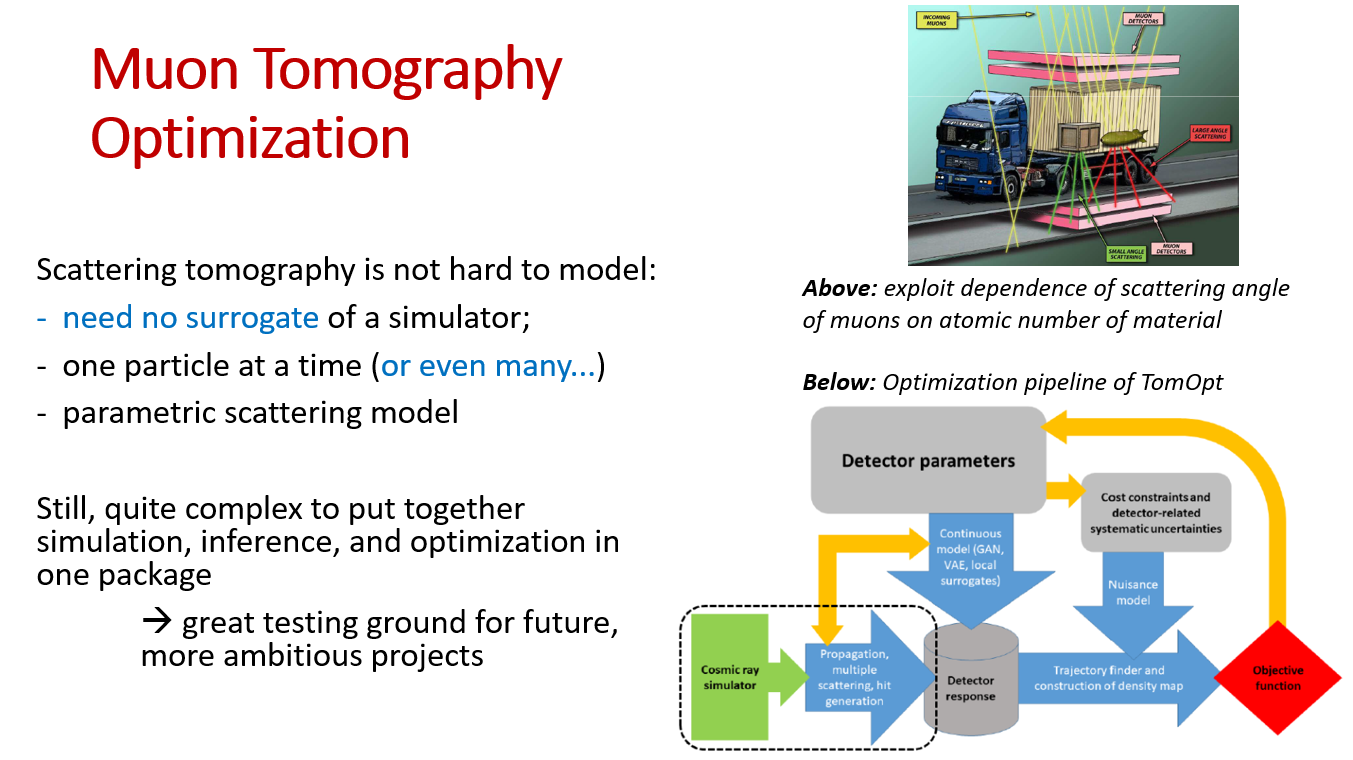
Finally I look at the future of particle physics and I point out a few challenges ahead of us.
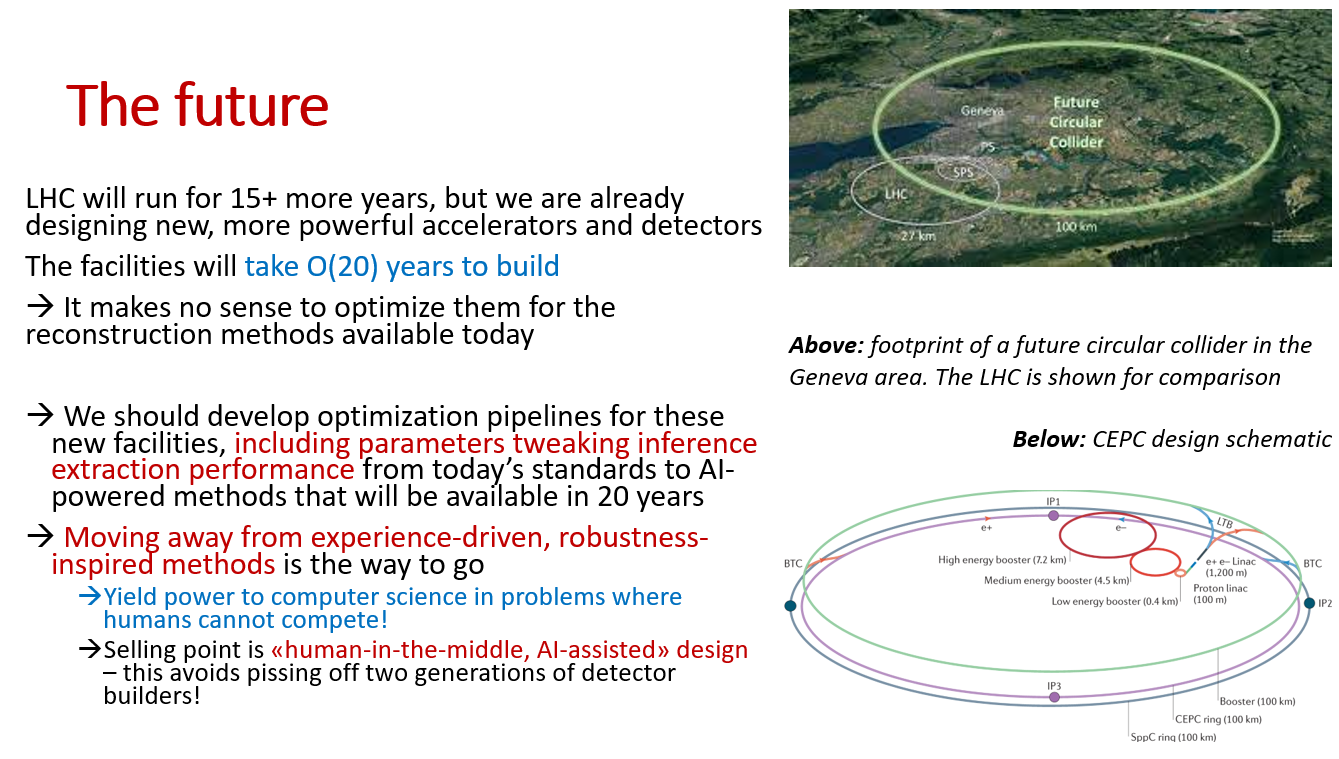
That is all - Lecture to be delivered in 1 h, so I have to hurry.
Comments