The campus of ICTS is very nice from an architectonic point of view, embedding nature in its buildings and trying to integrate the two realities. Below is a picture.
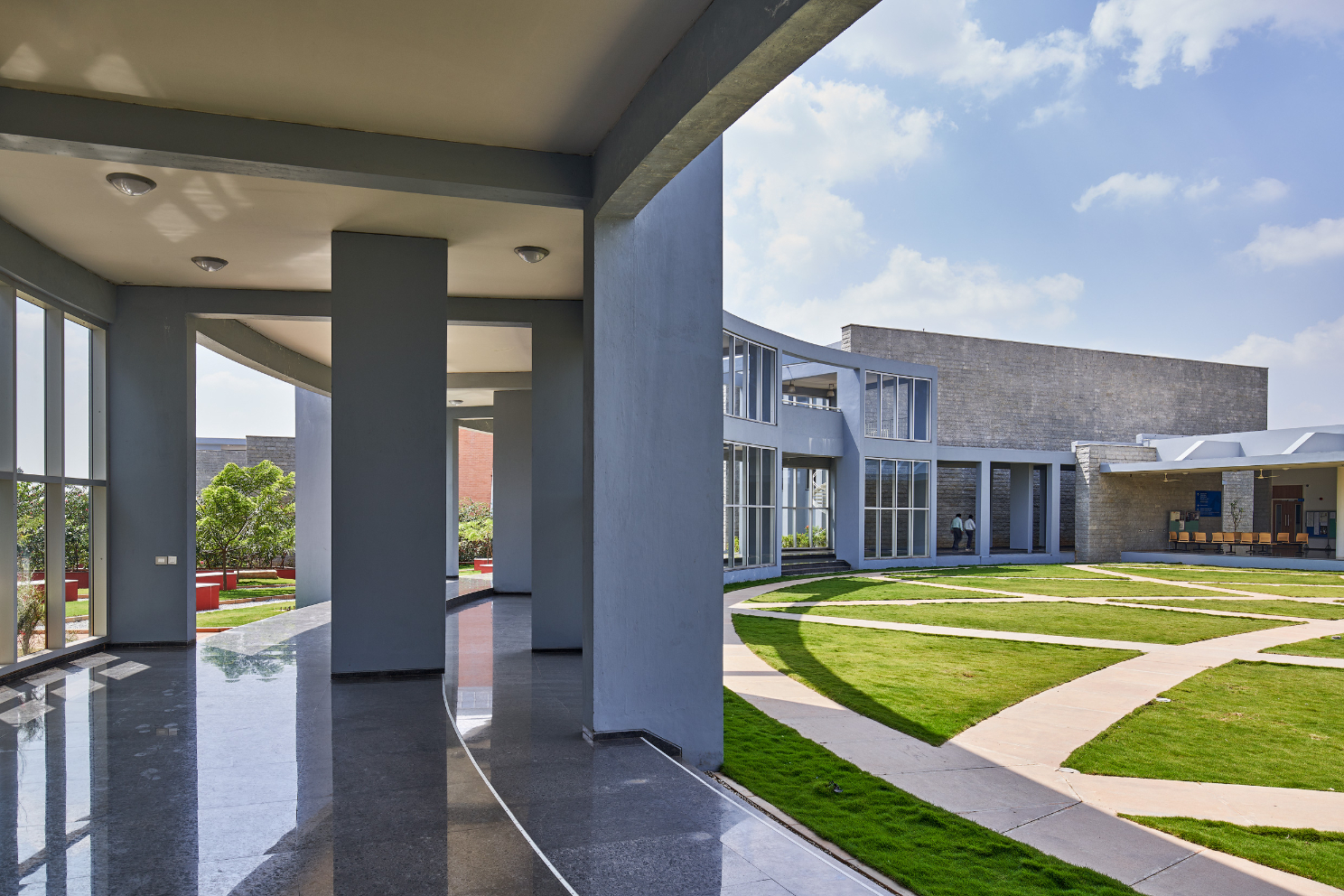
I visited ICTS to give 10.5 hours of lectures in Statistics for data analysis (plus a 3-hours long session on differentiable programming) at a Ph.D. School organized by the indian HEP community, in particular some colleagues who participate in the CMS experiment at CERN. The school had 50 participants selected from over 200 applicants. They were mostly PhD students and post-docs, with a mix between HEP, astro-HEP, and phenomenology. They seemed all very motivated to learn about machine learning (the main topic of the school, named in fact ML4HEP), but would they be interested in the boring lectures I had prepared for them?
The response will be gauged also by a poll, run after the school ends, but I already have some feedback from many of the students. These poor things had to sit through six hours of frontal lectures every day for an extended period of time. And whereas the machine learning lectures given by Sanmal Ganguly, Aishik Ghosh and others were often entertaining as they included time for performing exercises and testing ML methods, my lectures were objectively harder to digest, because of the material they focused on.
Still, the students seemed to enjoy the material I covered. I tried to include several examples of how the theory of estimators translates in practice in procedures, tests of coverage, comparisons between methods. I did have some 12 examples with code, which I provided although I challenged them to come up with their own solution to some of those. In the end, I suspect that not all of the students can be possibly interested in making the extra effort to study the material offline, but those who will are no doubt going to gain some insight in the topic.
After all, the material I used in my lectures is stuff that has been refined and tuned through a decade of courses in Padova and abroad. If I compare it to what I presented the first time I was invited to a PhD school - in Engelberg, 2012 - the quality has improved by a whole lot. That time I really did a poor job, because I was unexperienced in giving lectures at the PhD level and I incorrectly thought they should be of very high level, so I totally missed the target - the students did not have a good background in Statistics and most of the material was way above their head; plus I had three times more stuff to cover than the time allowed. Oh well, we learn through trial and error, especially the latter.
Before I leave the topic of the material I covered, I will touch on one issue here, which I believe many of you might be oblivious of. This is the weighted average of two measurements that have a significant correlation in their uncertainties. It is a very useful thing to discuss in lectures about estimators, as experimentalists need quite often to combine two measurements together, and systematic uncertainties almost always have some part that is shared by the two.
So, would you be surprised if you had to average two estimates of a physical quantity, and the result of the average ended up laying outside the range spanned by the two inputs?
Say you want to average x1 = 10+-1.0 and x2 = 11+-0.3, where the two measurements have a r=0.5 correlation factor among them. Would you have questions if I told you that the best estimate, which combines the above information, is <x> = 11.08 ?
The apparent paradox can be shed easily if you consider that the large correlation makes the two results "go along" with each other - meaning that if you make an overestimate once, you are likely to make an overestimate also if you remeasure the quantity. So the true value likely lays on the side of the more precise measurement (in this case, 11+-0.3).
The matter is actually quite fascinating if you look at the maths in more detail - especially since there are many ways to express the experimental conditions in the covariance matrix of two measurements, and depending on exactly you do it you may get results that make more or less sense to the eye. The results above, though, are obtained with no assumptions on the form of the matrix, by just using the correlation coefficient rather than getting into the business of exactly specifying how the two variances of x1 and x2 "talk" to one another.
The important lesson is that even the simplest problem in Statistics can be easily mishandled, and knowing a bit of theory is really important. Which is what Physicists typically lack!
Apart from the lectures, I had some free time to visit the city. I was a bit frightened by the possible cultural shock of a country so unique as India (I had never been there before) - the most populous country in the world, with a large number of extremely poor citizens and thus vast disparity in wealth, a country with very old roots in clash with a push for modernity and globalization, and now a country offering itself as a leader of technology, with the recent moon landing crowning it as a future leader of space exploration.
But in the end, there was no cultural shock at all. Yes, the traffic is completely crazy. But you can move about rather easily anyway; I always felt safe, everybody speaks English, and contrarily to my expectations you do not get assailed by needy people asking for money. And I had a funny incident when I first took a taxi ride, in one of those motorbikes redressed as micro-vans they use to carry customers around. I had in mind an exchange rate of the Euro to Indian rupee which was 10 times in favor of the rupee (I had read it somewhere before the trip without paying much attention to it), so when I asked the price of the ride to a driver and he said it was 200 rupees, I thought it must be too much, and I bargained a ride for 150 with some resistance on his part. When we got to my destination, I gave him two 100-rupee notes, and he said he had no change. Feeling like he was trying to fool me a bit I felt a bit annoyed, and after unsuccessfully trying to find somebody who could give me change for my notes I offered the driver to pay 100 rupees plus the rest in US dollars. He did not know the exchange rate but agreed, and I then handed him the 100 rupees plus 6 dollars. This effectively amounted to three times what he had initially asked for... When I later realized it, I was happy I actually paid more for the ride, but ashamed for having bargained a smaller price at the start! We should not forget that this is the country of Karma: I got what I deserved!
Comments