It is no surprise that some women were getting radical prophylactic mastectomies if they were told they had a gene that was statistically linked to breast cancer. Statistics are a blunt instrument but a new deep learning model identifies imaging biomarkers on screening mammograms to predict a patient's risk for developing breast cancer with greater accuracy than traditional risk assessment tools. That means informed decisions and not panic caused by media accounts of complex science issues.
Currently available risk assessment models incorporate only a small fraction of patient data such as family history, prior breast biopsies, and hormonal and reproductive history. Only one feature from the screening mammogram itself, breast density, is incorporated into traditional models.
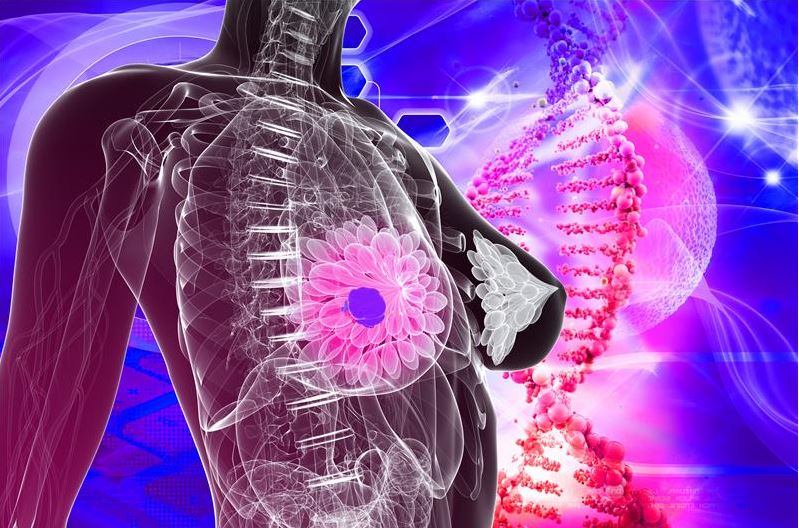
Image: Investigación y Desarrollo
"Why should we limit ourselves to only breast density when there is such rich digital data embedded in every woman's mammogram?" asked senior author Constance D. Lehman, M.D., Ph.D., division chief of breast imaging at Massachusetts General Hospital during the annual meeting of the Radiological Society of North America. "Every woman's mammogram is unique to her just like her thumbprint. It contains imaging biomarkers that are highly predictive of future cancer risk, but until we had the tools of deep learning, we were not able to extract this information to improve patient care."
Lamb and a team of researchers developed the new deep learning algorithm to predict breast cancer risk using data from five MGH breast cancer screening sites. The model was developed on a population that included women with a personal history of breast cancer, implants or prior biopsies.
The study included 245,753 consecutive 2D digital bilateral screening mammograms performed in 80,818 patients between 2009 and 2016. From the total mammograms, 210,819 exams in 56,831 patients were used for training, 25,644 exams from 7,021 patients for testing, and 9,290 exams from 3,961 patients for validation.
Using statistical analysis, the researchers compared the accuracy of the deep learning image-only model to a commercially available risk assessment model (Tyrer-Cuzick version 8) in predicting future breast cancer within five years of the index mammogram. The deep learning model achieved a predictive rate of 0.71, significantly outperforming the traditional risk model, which achieved a rate of 0.61.
Lamb said the new deep learning model has been externally validated in Sweden and Taiwan, and additional studies are planned for larger African-American and minority populations.
Comments