A new model used COVID-19 data for calibration and integrated SIR compartment modeling in time and a point process modeling approach in space–time, while also taking into account age-specific contact patterns. To do this, they used a two-step framework that allowed them to model data on infectious locations over time for different age groups.
Predicting the past is relatively easy but it is the best scholars have on the road to predicting future pandemics. In their computer simulation to assess the model’s performance, and in a case study of COVID-19 cases in Cali, Colombia, the model performed better when making predictions and provided similar results for past time points, compared with models commonly used in predictive modeling.
“The model’s features can help decision-makers to identify high-risk locations and vulnerable populations to develop better strategies for disease control,” says lead researcher André Amaral.
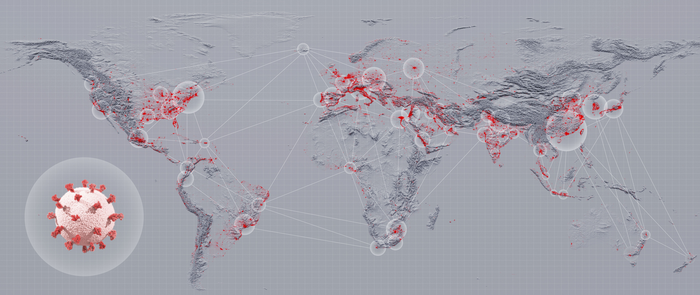
Credit: King Abdullah University of Science&Technology; Ivan Gromicho
Their model was able to account for different age classes and identify high-risk locations and vulnerable populations. The authors believe the model’s performance demonstrates the importance of quality and detailed data by location, time and population group to understand infectious disease dynamics, while highlighting the need to strengthen national surveillance systems to improve public health decision-making.
They believe it can also be used with any infectious disease that fits the compartment model assumptions, such as influenza.
“In future work, we might extend such an approach and use different temporal models to replace the SIR model. This would allow us to account for different epidemic dynamics and expand the number of scenarios that the model can be used for,” says Amaral.
Comments