Data from previous patients can help predict a new patient’s risk of complications after surgery, thanks to machine learning. Yet a framework for evaluating these algorithms must continue to evolve, and in a new op-ed, Tyler Loftus of University of Florida Health, Gainesville and colleagues propose six characteristics of ideal healthcare algorithms. To maximize its benefits, according to the researchers, an algorithm must be:
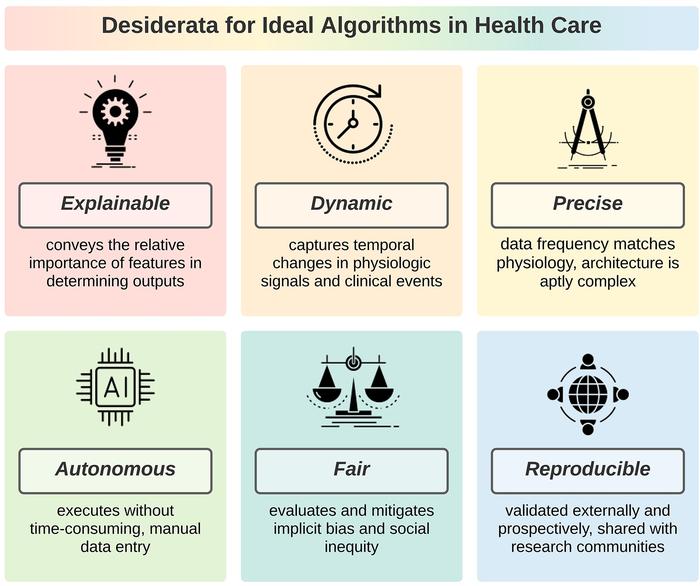
1. Explainable: able to clarify the relative importance of various characteristics of a patient and their medical condition in determining outcomes, without confusing association for causation
2. Dynamic: able to adjust predictions according to real-time changes in patient characteristics
3. Precise: able to take full advantage of data collected at a high enough resolution to robustly capture a patient’s changing condition
4. Autonomous: able to learn and return results with minimal human input
5. Fair: able to account for any implicit bias and social inequity
6. Reproducible: able to be shared widely with the research community for validation
To demonstrate this new framework, the researchers used it to evaluate eight algorithms that have been highly cited in academic literature. None fulfilled all six of the proposed characteristics, highlighting opportunities for improvement—especially in autonomy, fairness, explainability, and reproducibility.
Comments