How can we share 98% of our DNA with a chimpanzee and still be so different? One of the biggest biological surprises found in our genomes is that chimps, mice, and even flies don't differ very much from us in either number or types of genes. What makes the many diverse animal groups different is not what genes they have; the secret is in how those genes are used.
Something similar takes place inside ourselves: nearly every one of our cells carries the exact same DNA, and yet some cells transmit electrical signals in the brain, while others break down toxic compounds in the liver. How do you get such different cells from the same DNA? Again, the secret lies in how genes are regulated.
It should be no surprise then that gene regulation has been the subject of intense study. Most of these studies have focused on taking known genes and describing how they are regulated, but what biologists would really like to do is predict how an unfamiliar gene is controlled, simply by analyzing that gene's regulatory DNA. Once we can predict how genes are regulated, we're not far away from being able to design new regulatory DNA, which we can use to control the fate of stem cells, manipulate dosing in gene therapy, and design microbes that make better biofuels or degrade toxic waste. A new report in Nature describes an innovative new way to learn the logic of gene regulation.
Old Ways of Studying Gene Regulation
Two scientists at Washington University (full disclosure: one of them happens to be my boss, and the other sits across the lab from me), together with a physicist-turned biologist from The Rockefeller University, have come up with a new way to learn the logical rules of gene regulation.
To understand what this new approach does, it helps to look at how researchers have previously tackled this problem. One approach has been to study genes one by one, figuring out the operation of each region of regulatory DNA individually. For decades, this was the only feasible way to study gene regulation, and many great discoveries were made this way. But with 4000 genes in a simple bacterium, 6000 in brewer's yeast, and over 20,000 in humans, this one-by-one approach is dauntingly difficult. And not only is it daunting, but we are not really learning the rules of gene logic that effectively: after looking at 3000 yeast genes one by one, even the world's expert would still have a tough time telling you what level of gene expression the 3001st gene produces.
Another approach to studying gene regulation is has been much more focused on finding a way to predict gene regulation. This approach could be called the supercrunching approach, which takes advantage of the terabytes of data and large clusters of smoking hot CPUs that define today's post-genome era. In the supercrunching approach, researchers take large sets of data describing the behavior of thousands of genes at one time, called 'gene expression' data. This data essentially tells you the outcome of all of the unknown regulatory rules controlling these genes: which genes are on or off, and if they're on, how strongly that gene is expressed. You can take that data and, together with the DNA sequences of a regulatory region, plug it into a computer, use some fancy machine-learning algorithm and out pops a set of rules about gene regulatory logic: gene expression data + DNA sequence = rules governing gene expression.
In principle, this lets you do what the one-by-one approach doesn't: predict what level of gene expression to expect from that 3001st yeast gene. The computer model generates a set of rules, which you can use make predictions about a completely new gene. The problem is that so far, the predictions are not really that accurate. We still cannot look at the regulatory DNA sequence of gene and accurately predict how much the gene will be expressed.
Nature is Undersampling the Possibilities
The researchers at Washington University took a step beyond the normal supercrunching approach. They argue that one reason why the supercrunching approach has not been very successful is because of undersampling - there are simply not enough genes in a real genome to enable machine-learning algorithms to get handle on the gene regulation rules, and so supercrunching has failed. So what do you do if there aren't enough real regulatory regions in a genome? You make fake ones, which is exactly what this group of scientists did.
It may seem surprising to you that the 6000 genes in the brewer's yeast genome (yeast is the model system almost always used in this research) aren't enough to learn the rules of gene logic, but for each 'rule' of gene regulation, you only have at most a few dozen or a maybe a hundred instances in the genome. For example, there may be only a few dozen genes that are controlled by the regulatory proteins Gcr1 and Mig1, so if you're interested in the logic by which Gcr1 and Mig1 regulate their target genes, you don't have many examples to go on. To learn the logic underlying those genes regulated by Gcr1 and Mig1, you need many, many more examples.
To create those examples, the researchers stitched together bits of regulatory DNA in random combinations. There is a chunk of regulatory DNA that interacts with the regulatory factor Mig1, and a different bit that interacts with Gcr1, and you can place these two chunks of regulatory DNA together in random ways in the regulatory region upstream of a gene:
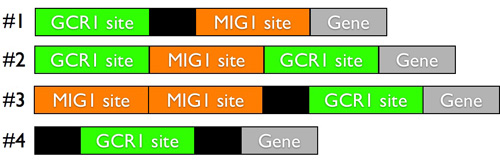
With our current understanding, we have very little ability to predict what level of gene expression you get from these random combinations of regulatory DNA.
To get that predictive power, the researchers built over 400 random combinations, and measured the resulting gene expression. Just as in the classic supercrunching approach, they now had a set of gene expression data from which they could infer the logical rules governing how Mig1 and Gcr1 regulate genes. And instead of a few dozen examples of Mig1/Gcr1 regulation found in the yeast genome, they had hundreds.
These scientists also made another improvement on the classic supercrunching approach: instead of employing some of the black-box, supercrunching machine-learning algorithms typically used to infer the regulatory rules, they used a physical model based on thermodynamics. Thus they were able to come up with regulatory rules which made physical sense, with some connection to the real behavior of the proteins and stretches of DNA found in the cell.
Once they used thermodynamics to infer gene regulatory logic from their gene expression data, the scientists put their regulatory rules to the test. They made completely new combinations of Mig1 and Gcr1 regulatory DNA, combinations for which they had no previous data. The researchers used their model of gene regulation to predict what the expression from these new, untested regulatory sequences would be. After making the predictions, they did the real experimental test, and found that, by using their inferred rules of regulatory logic, they were more than twice as accurate as the classic supercrunching models.
By being able to use these regulatory rules to accurately predict gene regulation, you can now look at an unstudied gene in the yeast genome and have a good idea of how that gene will be regulated. Applying this technique to the human genome in the future, we ought to be able to gain a fairly good grasp of how thousands of human genes are regulated, without having to study them in detail, one-by-one.
More importantly, this work lays the groundwork for much better gene therapy. For instance, cystic fibrosis patients have a mutation that breaks a critical gene. By supplying these people with with an unbroken copy of that gene, we may be able to cure the disease. But if that unbroken copy is mis-regulated, the patient gets the wrong dose of the gene, which perhaps ends up expressed in the wrong cell type, and thus the treatment is likely to fail. To make gene therapy successful, the unbroken copy will need to be under the control of the proper regulatory DNA that ensures the correct dosing of the gene: it needs to be expressed in the right place and at the right level. Obviously our chances of achieving successful gene therapy are much greater if we can design regulatory DNA to any specification we want.
They other key lesson from this research is that, even with gobs of computing power and large sets of data, it is important to recognize that nature may be undersampling the possibilities; to understand how the world works, we need to sometimes sample beyond nature.
Front page photo of microprocessor by Andrew Dunn, via the Wikipedia Commons.
Comments