For a human, knowing the difference between the "charge" of a battery and being charged in a crime is easy. Any three-year-old can look at a cartoon of a chicken and say "That's a chicken" but for computers those are still daunting tasks.
Obviously language is easier than visual recognition and linguists and programmers have spent 50 years trying to program semantics as software. While IBM's Jeopardy-winning Watson system and Google Translate are high profile, successful applications of language technologies, the humorous answers and mistranslations they sometimes produce are evidence of the continuing difficulty of the problem: that computers lack a lifetime of experience. Using the context in which a word is used, an intrinsic understanding of syntax and logic, and a sense of the speaker's intention, we can intuit what another person is telling us and programs cannot.
"In the past, people have tried to hand-code all of this knowledge," explains Katrin Erk, a professor of linguistics at The University of Texas at Austin focusing on lexical semantics. "I think it's fair to say that this hasn't been successful. There are just too many little things that humans know."
Other efforts have tried to use dictionary meanings to train computers to better understand language, but these attempts have also faced obstacles. Dictionaries have their own sense distinctions, which are crystal clear to the dictionary-maker but murky to the dictionary reader and no two dictionaries provide the same set of meanings.
Watching annotators struggle to make sense of conflicting definitions led Erk to try a different tactic. Instead of hard-coding human logic or deciphering dictionaries, why not mine a vast body of texts (which are a reflection of human knowledge) and use the implicit connections between the words to create a weighted map of relationships — a dictionary without a dictionary?
"An intuition for me was that you could visualize the different meanings of a word as points in space," she said. "You could think of them as sometimes far apart, like a battery charge and criminal charges, and sometimes close together, like criminal charges and accusations ("the newspaper published charges..."). The meaning of a word in a particular context is a point in this space. Then we don't have to say how many senses a word has. Instead we say: 'This use of the word is close to this usage in another sentence, but far away from the third use.'"
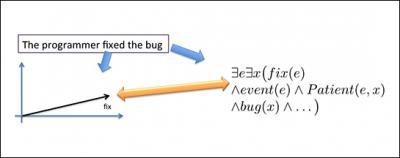
A sentence is translated to logic for inference with the Markov Logic Network and its words are translated to points in space. Here "fix" should be close to "correct" and far away from "attach." Credit: Katrin Erk, The University of Texas at Austin
To create a model that can accurately recreate the intuitive ability to distinguish word meaning requires a lot of text and a lot of analytical horsepower.
"The lower end for this kind of a research is a text collection of 100 million words," she explained. "If you can give me a few billion words, I'd be much happier. But how can we process all of that information? That's where supercomputers and Hadoop come in."
Erk initially conducted her research on desktop computers, but around 2009, she began using the parallel computing systems at the Texas Advanced Computing Center (TACC). Access to a special Hadoop-optimized subsystem on TACC's Longhorn supercomputer allowed Erk and her collaborators to expand the scope of their research. Hadoop is a software architecture well suited to text analysis and the data mining of unstructured data that can also take advantage of large computer clusters. Computational models that take weeks to run on a desktop computer can run in hours on Longhorn. This opened up new possibilities.
"In a simple case we count how often a word occurs in close proximity to other words. If you're doing this with one billion words, do you have a couple of days to wait to do the computation? It's no fun," Erk said. "With Hadoop on Longhorn, we could get the kind of data that we need to do language processing much faster. That enabled us to use larger amounts of data and develop better models."
Treating words in a relational, non-fixed way corresponds to emerging psychological notions of how the mind deals with language and concepts in general, according to Erk. Instead of rigid definitions, concepts have "fuzzy boundaries" where the meaning, value and limits of the idea can vary considerably according to the context or conditions. Erk takes this idea of language and recreates a model of it from hundreds of thousands of documents.
Say That Another Way
So how can we describe word meanings without a dictionary? One way is to use paraphrases. A good paraphrase is one that is "close to" the word meaning in that high-dimensional space that Erk described.
"We use a gigantic 10,000-dimentional space with all these different points for each word to predict paraphrases," Erk explained. "If I give you a sentence such as, 'This is a bright child,' the model can tell you automatically what are good paraphrases ('an intelligent child') and what are bad paraphrases ('a glaring child'). This is quite useful in language technology."
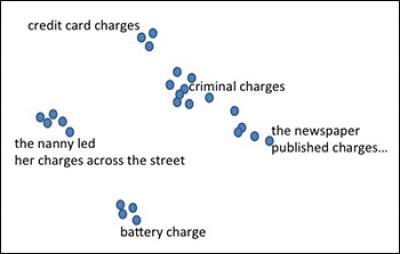
A "charge" can be a criminal charge, an accusation, a battery charge, or a person in your care. Some of those meanings are closer together, others further apart. Credit: Katrin Erk, The University of Texas at Austin
Language technology already helps millions of people perform practical and valuable tasks every day via web searches and question-answer systems, but it is poised for even more widespread applications.
Automatic information extraction is an application where Erk's paraphrasing research may be critical. Say, for instance, you want to extract a list of diseases, their causes, symptoms and cures from millions of pages of medical information on the web.
"Researchers use slightly different formulations when they talk about diseases, so knowing good paraphrases would help," Erk said.
In a paper to appear in ACM Transactions on Intelligent Systems and Technology, Erk and her collaborators illustrated they could achieve state-of-the-art results with their automatic paraphrasing approach.
Recently, Erk and Ray Mooney, a computer science professor also at The University of Texas at Austin, were awarded a grant from the Defense Advanced Research Projects Agency to combine Erk's distributional, high dimensional space representation of word meanings with a method of determining the structure of sentences based on Markov logic networks.
"Language is messy," said Mooney. "There is almost nothing that is true all the time. "When we ask, 'How similar is this sentence to another sentence?' our system turns that question into a probabilistic theorem-proving task and that task can be very computationally complex."
In their paper, "Montague Meets Markov: Deep Semantics with Probabilistic Logical Form," presented at the Second Joint Conference on Lexical and Computational Semantics (STARSEM2013) in June, Erk, Mooney and colleagues announced their results on a number of challenge problems from the field of artificial intelligence.
In one problem, Longhorn was given a sentence and had to infer whether another sentence was true based on the first. Using an ensemble of different sentence parsers, word meaning models and Markov logic implementations, Mooney and Erk's system predicted the correct answer with 85% accuracy. This is near the top results in this challenge. They continue to work to improve the system.
There is a common saying in the machine-learning world that goes: "There's no data like more data." While more data helps, taking advantage of that data is key.
"We want to get to a point where we don't have to learn a computer language to communicate with a computer. We'll just tell it what to do in natural language," Mooney said. "We're still a long way from having a computer that can understand language as well as a human being does, but we've made definite progress toward that goal."
Comments