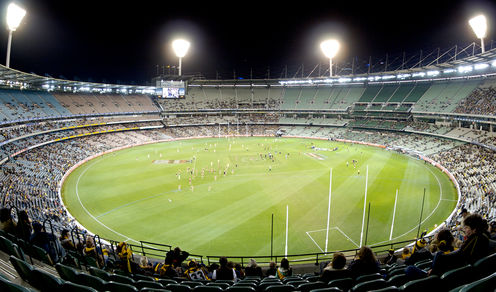
So professional clubs are constantly on the lookout for tools that can help turn these data into usable and meaningful information.
One such tool gaining popularity is machine learning. Put simply, machine learning is a form of artificial intelligence, whereby computers are able to learn without being explicitly programmed by a human operator.
What makes machine learning algorithms so useful is their ability to be trained on large pre-existing data sets. These trained algorithms can be used to identify potentially complex yet meaningful patterns in the data, which then allows us to predict or classify future instances or events.
Machine learning approaches often outperform traditional statistical techniques, which are largely incapable of accounting for the dynamic and almost random patterns in so much of the data obtained from sport.
A game of football
Consider a typical game of elite Australian Rules football. During any match played in the Australian Football League (AFL), sources of information relating to player movement and performance are available in near real time to coaches and support staff.
Despite access to this information, the ability of coaches to observe, process and evaluate the actions of 18 players on different areas of the field is limited. And that doesn’t even include the opposition. As humans, coaches simply do not possess the capacity to undertake such a task successfully.
Don Norman summarizes this predicament in his book Things That Make Us Smart: Defending Human Attributes in the Age of the Machine. He says the power of the unaided human mind is overrated. This is due partly to our inability to overcome inherent limitations in areas such as memory and conscious reasoning:
Human memory is well tuned to remember the substance and meaning of events, not the details […] Humans can essentially attend to only one conscious task at a time. We cannot maintain attention on a task for extended periods.
In the sporting context, coaches are therefore limited with respect to their cognitive abilities. This can only be improved by developing external aids, such as machine learning, to enhance these skills.
Suppose we consider a short five-second period of play within an AFL match, featuring multiple players each undertaking different movement patterns and performing various skilled actions.
Try and keep your eye on all the action in the grand final highlights.
This brief section of the game could potentially be construed differently by multiple coaches working within a single team, depending on cognitive biases, including previous experience, prejudices and individual personality traits.
Machine learning thus has a place in providing rapid, objective evidence obtained from data in order to help inform coach decision-making.
The science in sport
But what about the scientific evidence for this approach? The peer-reviewed sports science literature is actually full of successful applications of machine learning to sport.
Examples from biomechanics, in particular, show extensive use of machine learning. Notably, pattern recognition algorithms have been developed to identify individual athlete movement sequences in a variety of different sports.
In soccer, machine learning match analysis has been used to identify the conservative strategies of away teams competing in the English Premier League. It has also been used to discover the optimal methods by which teams obtain a shot on goal, or return the ball in tennis.
Machine learning has been used to predict the behavior of individual athletes, such as cricket bowlers in the Indian Premier League, and team performance in the Asian Games based on factors varying from athlete age and experience levels to national social-cultural factors.
Researchers from the University of Coimbra, in Portugal, successfully implemented a suite of machine learning algorithms to identify talented basketball players based on their psychological characteristics and practice history.
A team from Deakin University developed a set of rules to explain the physical characteristics most strongly linked with Australian Rules football draft success.
A body of work focusing on automated classification of human movements including kicking, running and jumping using wearable technologies has also emerged.
Machine learning is also being used to help predict the return-to-play time for soccer players following an injury and in selecting the appropriate balance of batsmen and bowlers in a cricket match.
Machine learning vs. human coaching
Improvements in technology and machine learning continue to progress the field towards artificial intelligence and real-time use in sport. But is it possible that computers will ever replace the coach?
Well, in some ways, they already have. Many elite sporting clubs already set specific thresholds for athletes during training. These are based on perceived reductions in performance or increases in injury risk if this threshold is overcome.
The judgement on what is appropriate treatment of the athlete is made solely by a computer-based analysis of data collected in the field. For the moment, at least, the decision on whether to act or not on this information still remains with the coach.
But in future that may well change.
Sam Robertson is Senior Research Fellow Victoria University/Western Bulldogs at Victoria University. This article was originally published on The Conversation. Read the original article. Top image: AFL match at the MCG in Melbourne. Sascha Wenninger, CC BY-SA
Comments