It means just what it reads like. Statistics can be used for anything. Coronavirus created a difficult problem for disease epidemiologists in that epidemiology had been overrun by claims about any chemical being linked to "greater risk" of some disease while any food can be linked "increased" longevity. Those claims were not fabricated by journalists, groups from International Agency for Research on Cancer (IARC) to the U.S. National Institute of Environmental Health Sciences (NIEHS) have intentionally manipulated results to achieve cultural outcomes, like lawsuits on weedkillers or popular foods. Harvard School of Public Health pushes out terrible "food frequency questionnaire" papers every month, and calls them science.
Beware the premature consensus
So when a real problem came along - SARS-CoV-2 - can we trust recommendations from experts when those collecting the data know how flawed it is while those making models using the data think they are doing science? In a consensus, the average man has fewer than two testicles but such ridiculous averaging is not how policy decisions should be made. Policymakers, from the U.N. to the U.S., often do rely on reaching such a consensus, but why should models that are not very good be allowed to offset ones done with more rigor?
A new paper hopes to clear up some of the confusion by outlining best practices when synthesizing results from multiple modeling groups. Peer review is not much help after the fact, as has been shown in numerous cases. Ridiculous papers about homeopathy - except they call it a u-shaped curve when it is endocrine disruption - can pass peer review at the NIEHS fanzine Environmental Health Perspectives the same way an astrology paper can pass peer review at Astrology Monthly; you can't get into their system without being a believer.
But no one can see your flaws like a competitor, and competition is likely the answer here too.
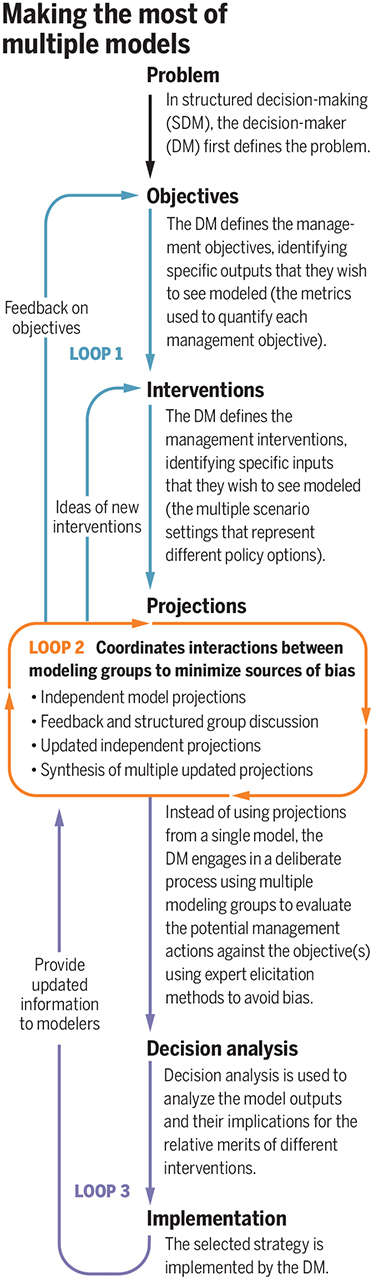
GRAPHIC: N. CARY/SCIENCE
The first challenge will be the hardest part; everyone can spot the flaws in someone else's method but defend their own shortcuts. Synthesizing multiple model methods means looking at why models disagree - not excluding studies that disagree, as IARC does - and then refining them based on that feedback. Since academics are often in competition with each other(1) a culture shift to being open to change is needed. In the current academic climate, concessions are a sign of weakness (2) but this is not some unimportant trifle like defending a statistical correlation of IQ to virtual pollution such as PM2.5, COVID-19 has actual real-world implications.
Embrace uncertainty - because the precautionary principle is where good data go to die
Policymakers deal in a world of uncertainty so it is actually okay to create a projection for each risk management strategy - and acknowledge the flaws.(3) Fundamentalism is the enemy. Sure, Paul Krugman can write his dreary economic dirges in the New York Times but no one gives the guy whose biggest economic success was Enron real money.
In the real world, we don't have a speed limit of 5 miles per hour even though it would prevent all traffic deaths to lower it. It is equally ridiculous to claim that the United States should remain shut down until a virus in the same family as the common cold can no longer be detected anywhere - that will never happen. But a blanket consensus brought by averaging would mean Montana has to suffer economic hardship because residents of Manhattan chose to cluster on an island a few miles long and their mayor made cars so expensive that the most vulnerable people have to ride in crowded disease hotbeds from place to place.
A premature consensus created by hand-selected insiders is not a valid consensus.(4) It can lead to hasty decisions that kill businesses and cost trillions of dollars. Uncertainty, which includes acknowledging what we don't know, is also the way the models get improved. Picking a model and then moving economies based on that could be a recipe for disaster.
Few corporate journalists will say so, but coronavirus is actually a good test case for better adaptive management to disease risk because it is not deadly. I know, I know, I am going to be in your Twitter mentions and you will threaten to end my career for writing that, but it's true. There was no meltdown two years ago when 60,000 Americans died from flu so it is a little hypocritical to cancel culture everyone who notes that the same people at greatest risk of respiratory distress from COVID-19 were also at risk from influenza this year. Even kids die from flu but a year ago half of Americans said they didn't even consider bothering with a flu vaccine.(5)
We can't make decisions based on epidemiologists who get a lot of retweets either. Professor Neil Ferguson claimed 500,000 Brits would die unless they went into lockdown until June - and then had his married lover sneaking over to see him in violation of the policies he got England to implement. He didn't believe his own model, how can the British public? But if her husband was an expert in modeling and conceded Professor Lockdown was still right, that would really mean something.
The kind of meta-analysis approach in the paper is not new but because these techniques are also often abused by the same epidemiologists who told us bacon is as hazardous for your health as asbestos, it can still be hard to trust. But we do have to pick a spot and start somewhere, even without all of the data. After that, you can converge on a better answer.
So if I were on President Trump's advisory panel I would recommend some cranky old expert in statistics to oversee any recommendations involving models. Statistics experts can see all of the flaws in the numerical methodology epidemiologists use, they never believe the data or the modeler without fact-checking. They don't believe anything until the method is proven valid.
I'm a statistician. My motto is 'I haven't read your paper yet but I'm virtually certain your methods are flawed&your results are wrong.'
— Stephen John Senn (@stephensenn) April 9, 2014
NOTES:
(1) This century brought a desire to get one of those high-paying tenure jobs and schools are pushing out 6X as many PhDs as there are university jobs - so now only 10 percent of R01 applicants will get a grant.
(2) The same way few are willing to apply for a grant for a bold experiment that might fail. If you fail, your career could be over. It is much safer to create a modest experiment likely to succeed, which is why academia has become more incremental.
(3) The only problem may be when politicians say there can be "no risk" as part of their political theater, like often happens with food, energy, chemicals, and medicine, but that lack of logic is not the problem for groups creating the models.
(4) If only epidemiology papers carried the kinds of warnings they set out to get placed on useful products using nothing more valid than cell cultures, studies in rats, and breezy correlation:
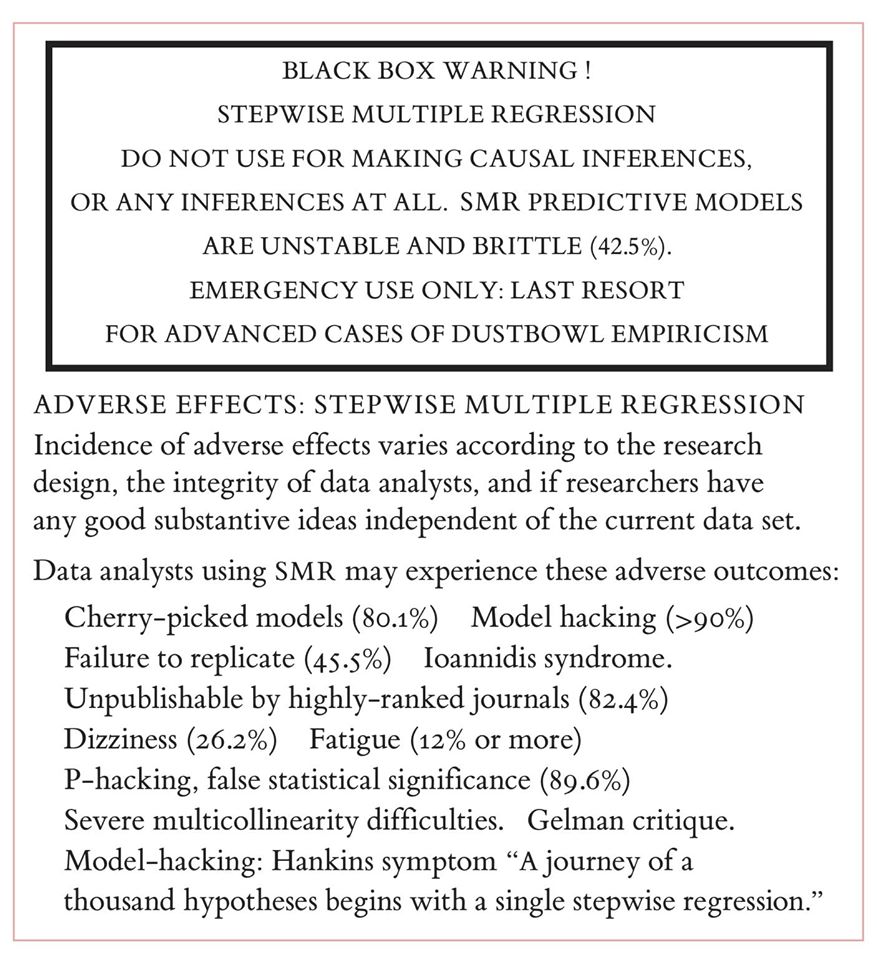
(5) In a recent survey, 25 percent still said they wouldn't take a vaccine to prevent COVID-19.
Comments