Among the many things I had marked down for subsequent discussion in this blog is a very interesting statistical "paradox", which highlights the opposite conclusions that a researcher might reach if she were to adopt either of the two "schools of thought" of theoretical Statistics. Examples of this kind are important because we use statistical procedires for most of our scientific results, and as scientists we are accustomed to think at the data as giving unequivocal answers. Statistics, however, messes things up, since the experimenter's choices have an impact on the final result.
The paradox in question is called "Jeffreys-Lindley" and arises in cases when one tests a hypothesis with a high-statistics dataset. Rather than discuss the generalities, I think it is most proficuous if I go straight to a practical example, leaving the discussion to the end.
Imagine you analyze data from one of the LEP experiments: these were four detectors (L3, ALEPH, DELPHI, OPAL) that looked at electron-positron collisions at a center-of-mass energy of 91 GeV. Such collisions are very clean -the projectiles are structureless, and their interaction is rare enough that only one collision every few second is produced. Further, the final state has zero total electric charge, and the "hermeticity" of detectors for e+e- collisions allow the detection of a large fraction -close to 100%- of the charged particles that get produced. So the researcher might decide to study with high precision the possible charge bias of her detector by just counting tracks with positive and negative curvature. The track curvature is due to the strong axial magnetic field existing at the center of these detectors (except L3, where the field was absent).
After counting many tracks, a result significantly different from zero would clearly indicate that the detector or the software have a different efficiency to reconstruct tracks curving in one or the opposite direction under the action of the axial magnetic field.
Let us say that she collects n_tot=1,000,000 tracks, and gets n_pos=498,800 positive and n_neg=501,200 negative tracks. The hypothesis under test is that the fraction of positive tracks is R=0.5 (i.e., no charge bias), and let us add that she decides to "reject" the hypothesis if the result has a probability of less than 5%: this is referred to as a "size 0.05" test.
A Bayesian researcher will need a prior probability density function (PDF) to make a statistical inference: a function describing the pre-experiment degree of belief on the value of R. From a scientific standpoint, adding such a "subjective" input is questionable, and indeed the thread of arguments is endless; what can be agreed upon is that in science a prior PDF which contains as little information as possible is mostly agreed to be the lesser evil, if one is doing things in a Bayesian way.
A "know-nothing" approach might then be to choose a prior PDF by assigning equal weights to the possibility that R=0.5 and to the possibility that R is different from 0.5. Then the calculation goes as follows: the probability to observe a number of positive tracks as small as the one observed can be written, with x=n_pos/n_tot, as N(x,σ), with σ^2=x*(1-x)/n_tot (we are in a regime where the Gaussian approximation holds, and the variance written above is indeed the Gaussian approximation to the variance of a Binomial ratio). N(x,σ) is just a Gaussian distribution of mean x and variance σ^2.
If we have a prior, and the data, we can use Bayes theorem to obtain the posterior PDF: in formulas,

(If the above does not make sense to you, you might either want to check Bayes' theorem elsewhere, or believe the calculation as is and try to make sense of the rest of this post -the math is inessential).
From the above value, higher than the size α=0.05 and actually very close to 1, a Bayesian researcher concludes that there is no evidence against R=0.5; the obtained data strongly support the null hypothesis.
Frequentists, on the other hand, will not need a prior, and they will just ask themselves how often a result as "extreme" as the one observed arises by chance, if the underlying distribution is indeed N(x,σ^2), with x=0.5 and σ^2=x*(1-x)/n_tot as before. One then has:
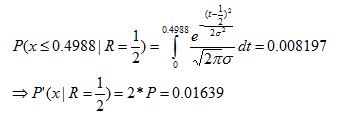
(we are multiplying by two the probability in the second row, since if H0 holds we are just as surprised to observe an excess of positive tracks as a deficit!).
From the above expression, the Frequentist researcher concludes that the tracker is indeed biased, and rejects the null hypothesis H0, since there is a less-than-2% probability (P'<α) that a result as the one observed could arise by chance! A Frequentist thus draws, strongly, the opposite conclusion than a Bayesian from the same set of data. How to solve the riddle ?
There is no solution: the two statistical methods answer different questions, so if they agreed on the answer it would be just by sheer chance. The strong weight given by Bayesian on the hypothesis of a unbiased tracker is questionable, but not unreasonable: the detector was built with that goal. Notice, however, that it is only the high-statistics power of the data that allows the contradiction with a Frequentist result to emerge. One might decide to play with the prior PDF and make it such that the Bayesian and Frequentist answers coincide; but what would we learn from that ?
Comments