The book comes in soft cover (I am unaware if a hardcover will also be available any time soon), and is printed on nice and good-smelling acid-free paper. This might look like a detail to you, but a good part of my judgement about a book comes from the way it smells ;-). The book costs $89 at WILEY, and you can get a good preview of the material and sample chapters at this site.
The cover shows a CMS heavy-ion event display overlaid to a few typical graphs of multivariate techniques. I should mention that the subtitle reads "Fits, Density Estimation and Supervised Learning". Indeed, the book is geared toward multivariate techniques in data analysis, and cannot be mistaken for a general-purpose book on statistical techniques; despite that, the authors have made an effort to insert in the first two chapters (ch.2 and ch.3, as the first is an introduction) some reminder of the most important techniques. Of course one cannot imagine that this is more than a quick reminder aimed at readers who already know the topics dealt with there: goodness-of-fit tests, which are the main subject of chapter 3, are done with in 15 pages; confidence intervals, in chapter 2, occupy even a few less.
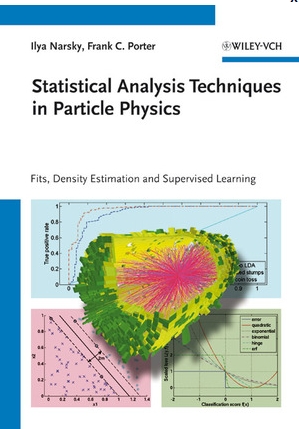
Every chapter is complemented with a few exercises and a selected list of references. I am not a big fan of books that give you work assignments without offering a solution at the back, or at least some trace of the solution; I hope authors will make an effort to provide worked out solutions to at least a sample of the exercises in their site. That would be a great addition to the material they offer.
All in all, I believe this is a very useful book for researchers who wish to learn more about the techniques that have become available in the course of the last 20 years to achieve more powerful inference from their data. Not knowing what are kernel estimation, bootstrap, or random forests has become increasingly embarassing if you work in the field.
Comments