A further distinction among calorimeters for particle physics is the one concerning the kind of particles these devices aim to measure. Electromagnetic calorimeters target electrons and photons, and hadronic calorimeters target particles made of quarks and gluons. Here I will discuss only the latter, which are arguably more complex to design.
Smashing protons
If you take a proton endowed with a kinetic energy of 100 GeV (enough to make it a very interesting particle to detect and measure well, in modern-day particle physics experiments), and let it hit a block of iron thick enough to stop the proton along with all the other particles it produces as it smashes onto some iron nucleus, will the iron block get red hot ? Not at all - it will only raise its temperature by some picodegrees (I did the calculation long ago and I remember some 4*10^{-12} degrees Celsius increase, but I forgot the weight I had assumed for it). Measuring such a tiny heat release would therefore be a very silly way to try and infer the energy of the incident proton.
Calorimeters for particle physics work by another principle. We know that when a proton or another hadron (a particle made of quarks and gluons; besides the proton, this category includes atomic nuclei, as well as the neutron, and many unstable subatomic particles with names such as pions, kaons, hyperons, etcetera) impinges on dense matter it will eventually hit head on a nucleus.
When that happens, the strong interaction between projectile and target converts a good part of the projectile energy into new particles, and gives them significant kinetic energy. Those released particles, along with the slowed-down projectile and with fragments of the nucleus, are now loose in the material and produce further hard collisions, generating new secondary particles of progressively lower energy.
Eventually, the process peters out, and most of the produced particles stop in the material; a few however (typically, neutrinos and muons) whiz through and get lost at the far end of the block of matter. Because the multiplication process initiated by the incident proton continues until a "critical energy" is reached by the secondaries, the total number of produced particles turns out to be a good tracker of the incident proton energy: an almost linear relation between the proton energy and the number of secondary particles in the block of matter exists.
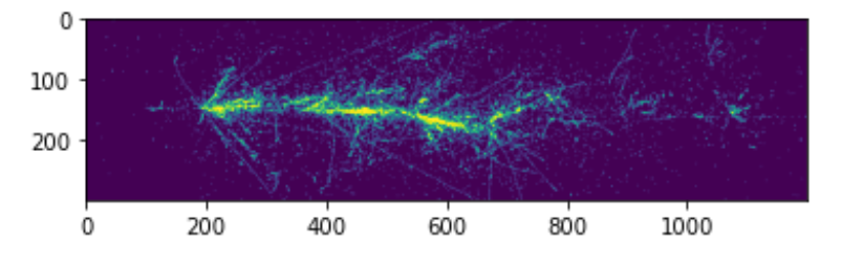
Above: Geant-4 simulation of a hadronic shower produced by a 100 GeV proton (impinging from the left) in a finely-grained block of lead tungstate. The scale is in millimeters.
The mentioned proportionality is at the core of the construction principle of calorimeters: If you can count the secondaries produced in the material, you can work out the energy of the particle(s) that hit it.
How hadron calorimeters are built
There are several ways to detect the passage of particles in a material, and most of them have been found suitable to design calorimeters. You can use a gas that gets ionized by the passage of the charged particles, and record how much ionization you got; or use transparent materials that release light when they are traversed by the particles, when you couple these to photomultipliers or other devices that count the amount of produced light; and so on. But one thing is clear: you need a lot of dense material to let the particles find nuclei along their path, lest you fail to catch them and lose them as they leave it at the back end.
In the past, hundreds of hadron calorimeters have been designed and constructed. Those specifically designed for collider detectors, that must record particles of very high energy, are big and bulky. The reason is that atomic nuclei are small, so you need to pack many big nuclei (heavy materials such as lead, iron, or uranium can be used) and compute how thick the device needs to be in order to completely absorb the highest incident particles you wish to measure. The length scale that you will then use is called interaction length, a distance that has the typical scale of a few inches. Then you have to consider that if on average a particle will hit a nucleus every interaction length, it will not immediately stop: you need some 10 interaction lengths or so to ensure that you do not lose a large fraction of the particles at the back end.
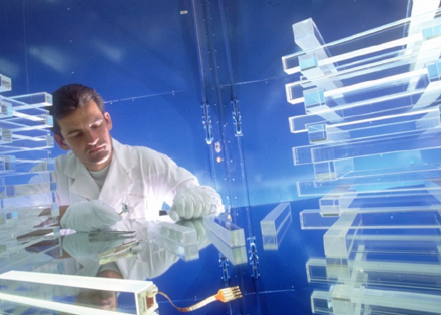
Above: lead tungstate crystals for the CMS EM calorimeter, in a testing lab
And then, the annoying thing about those heavy elements is that they are not transparent to light - so you need to figure out whether to insert scintillating crystals interspersed with thick absorbing layers, or whether instead to go for heavy transparent materials. One such material is lead tungstate, which can be manufactured in nice transparent crystals. The CMS electromagnetic calorimeter was built with that material - about 80,000 3-by-3-by-25 cm pieces of it: it provides excellent energy resolution because you collect information about all the secondary particles, and it is very dense, so you can build compact structures. You can of course use the same technology for hadron calorimeters, but - why should one do so?
Two groundbreaking elements
Indeed, while the CMS electromagnetic calorimeter was built with the goal of measuring with extreme resolution the energy of high-energy photons produced in the decay of Higgs bosons (a feat it indeed pulled off, allowing CMS to discover the Higgs boson in 2012), measuring the energy of particles in hadron calorimeters was never a task that collider detector designers got a headache about. The reason is that hadrons are typically produced in streams, collectively called hadronic jets.
Jet production is the phenomenon that takes place when a quark or a gluon, kicked out of the interior of a proton by the strong interaction produced in the collision (e.g. with another proton, at the LHC, or with an antiproton, in earlier colliders like the SppS or the Tevatron), fragments into anything from several to a few dozen hadrons. These particles (or the product of their decays, when they are too short lived to make it far away from the collision point) eventually hit the calorimeter together, and get measured together.
Until a couple of decades ago, hadron calorimeter designers only cared to measure the total energy of the jet, which tracks the energy of the original quark. So forget the fancy 3x3 cm^2 grid of lead tungstate cells: big blocks of material and no fine segmentation was invariably used. But then two groundbreaking things happened.
The first thing that revolutionized the idea of how hadron calorimeters should be designed, in high-energy colliders, was a realization of about 20 years ago, that if one could look inside the detailed structure of energetic jets, one had a chance to identify in there the decay of heavy particles, such as W and Z bosons, top quarks, and now Higgs bosons too (after we showed that the Higgs also exists). This completely changed the perspective of hadron collider physics: to search for the highest-energy phenomena, which might involve production of very heavy particles that could decay into W, Z, h bosons, one needed to get equipped with fine-grained calorimeters, which could evidence those particles inside the jets.
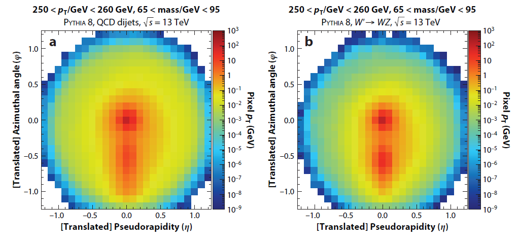
Above: the "image" of jet energy deposits in a granular calorimeter allows to distinguish, on a statistical basis, jets originated by strong interactions (left) from jets originated from the decay of heavy bosons (right).
The second realization came as a by-product of the small budget that CMS had allocated for the construction of its hadron calorimeter. The focus of discovering the higgs boson diverted funds to realizing a very performant electromagnetic calorimeter, and an excellent silicon tracking detector. But when the detector was eventually built, the resolution of the CMS hadron calorimeter ended up to be significantly worse than that of the CMS competitor at the LHC, ATLAS. To compete, CMS pulled off a wonderful study of the details of jet energy measurement, where by leveraging the strong points of the detector (in particular, its tracker and EM calorimeter, and the 4-Tesla solenoid), it could produce a detailed reconstruction of all the different particles inside the jets, hence allowing the tracker to measure the momentum of all charged particles and the EM calorimeter to measure the energy of all electromagnetic particles (electrons and photons) within each jet. This "Particle Flow" algorithm saved the day, and put back on par the performance of energy measurement in the two hadron calorimeters of the giant LHC competitors.
The future
Where does this leave us? In the future, we will need to build even more granular calorimeters for the higher-energy endeavours we have started to design. Of course, we will employ the cutting edge of electronics and material science to build these devices. But maybe we can also think at modifying our paradigms. In fact, another big thing has happened in the past 20 years - Artificial Intelligence (AI) is now impossible to ignore. Applied to the reconstruction problem of hadron collisions, AI allows to make sense of information that until now we have been neglecting. What do I mean by that?
The particle flow algorithm taught us that if we can zoom into the constituents of jets we improve the overall energy resolution of the calorimeter. To go further we must leverage the fact that different hadrons produce different physics processes when they hit nuclei. On average things look the same if we consider a proton, a kaon, or a pion hitting a nucleus with the same energy; and yet, these particles are made of different quarks, and have different sizes, and different interaction rates; the collision produces different number and species of secondaries. What if we could know exactly which particle traveled through a very highly granular calorimeter? We could improve our energy resolution by a large amount, but we could also improve our algorithms that distinguish jets produced by different quark flavours, opening up to more accurate studies of subnuclear physics.
One big question is thus the following: imagine we have unlimited funding, and imagine we can push the size of calorimeter cells read independently to be smaller than one cubic centimeter - let us say a cubic millimeter, e.g. That calls for the need to read out millions, if not billions of independent cells, but let us leave this aside for an instant. Would an AI algorithm be able to distinguish the different particles produced and traversing a calorimeter? How big would the discrimination power be? And what if the cells were twice as wide, or three times more... Where would the AI end up throwing its hands up and say "there is no discrimination possible between these particles, your calorimeter is too crappy"? Answering this question would set the target scale for building the calorimeters of the future.
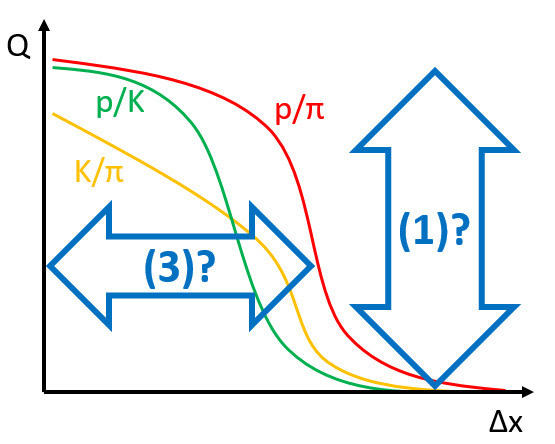
Above: hypothetical discrimination power curves of particle species as a function of the size of the calorimeter cells. There are two quantities of relevance: the scale at which discrmination is still possible (3), and the amount of discrimination achievable (1).
But there are more questions. One paradigm that is just waiting to be pulled down is the need for a light-weight tracking detector followed by a very thick calorimeter to measure particles in high-energy collisions. Think about it: it is like a digital answer, 0 - 1. Zero mass, maximum mass. Why do we rely on this kind of separation? Of course, we want to measure the trajectory of all charged particles through the small amount of ionization they leave when they kick electrons off atoms in a light medium. If we do so, we do not destroy the particles, and we get to fit nice helical trajectories in a magnetic field, obtaining precise estimate of the particles momenta. Then, the same particles can reach the thick calorimeters, where they get destroyed and their energy gets measured. The calorimeters can do the same trick to neutral particles as well (photons, neutrons, neutral kaons), so we get the best of both worlds...
Having a thicker tracking detector has been always dreaded by particle physicists: so much so, that one of the quality indicators was always the total amount of material that a particle would cross when going through them: the smaller, the better. That is because this minimizes the multiple scattering that the particle withstands, and also minimizes the chance of an infrequent nuclear interaction, which would destroy the particle and produce a mess of secondaries that our tracking algorithms would have trouble decrypting. But now we have AI...
Indeed, we can think of leveraging, rather than dreading, nuclear interactions in a tracking device, if we get information on the particle species from it. The 0-1 dychotomy can be overcome if we conceive a detector where an inner lightweight tracker made of silicon pixels does its job in the first ten inches or so, leaving way to a medium-thickness material where interactions can still be tracked, and particles followed through individually, but where information is generated from the different interaction properties of the diffeernt particles. Timing detection layers can add information here too. Then the thick calorimeter can do its job better, as the algorithms reading it out will be able to couple with more effect the particle identity information with the energy deposits.
While the above hybridization of tracking and calorimetry for future colliders is only an idea, we should not dismiss it. In fact, I have recently started to study precisely the above questions. A research grantee has recently joined my group, and will work with me and Nicolas Gauger's computer science group in Kaiserslautern (RPTU) to tackle these questions. Our group will also look at how such impossibly granular calorimeters could be read out effectively, and here the idea we have is to employ neuromorphic computing provided with nano-photonic devices embedded in the calorimeter, to produce high-level primitives on the detailed properties of the nuclear interactions locally happening in the material. A PhD student we hired in Padova jointly with Fredrik Sandin from the computer science group of Lulea Techniska Universitet has started working at this application...
Ultimately, we want to apply gradient-based optimization to these calorimeters: not only finding useful new ways to measure particles by overcoming past paradigms, but also making sure that we get the absolute best performance from them, by navigating through the high-dimensional space of design choices with differentiable models of the detectors, the data-generation and collection mechanisms, and the inferecne procedures. It is a grand plan, which I estimate will take all the time I have before my retirement, but it is certainly an exciting one for me!
So, if you are so interested in this research to have reached the end of this article, I invite you to contact me to see if we can collaborate! There are master theses I offer in Padova on these topics, but there may in the future also be PhD opportunities, for students with familiarity with machine learning and particle physics...
Comments