A new study finds those may be flipped. The distinction was never absolute anyway. Though it was only in 1996 that a computer beat a chess champion, computers beat lower quality players all of the time. And our memory may be better than we think, it is instead that the brain strategy for storing memories may lead to imperfect memories, while allowing it to store more memories easier than Artificial Intelligence.
Neural networks learn by tweaking the connections between neurons. Making them stronger or weaker, some neurons become more active, some less, until a pattern of activity emerges. This pattern is what we call "a memory". The AI strategy of mimicking neural networks is to use complex long algorithms, which iteratively tune and optimize the connections. The brain does it much simpler: each connection between neurons changes just based on how active the two neurons are at the same time. When compared to the AI algorithm, this had long been thought to permit the storage of fewer memories. But, in terms of memory capacity and retrieval, this wisdom is largely based on analyzing networks assuming a fundamental simplification: that neurons can be considered as binary units.
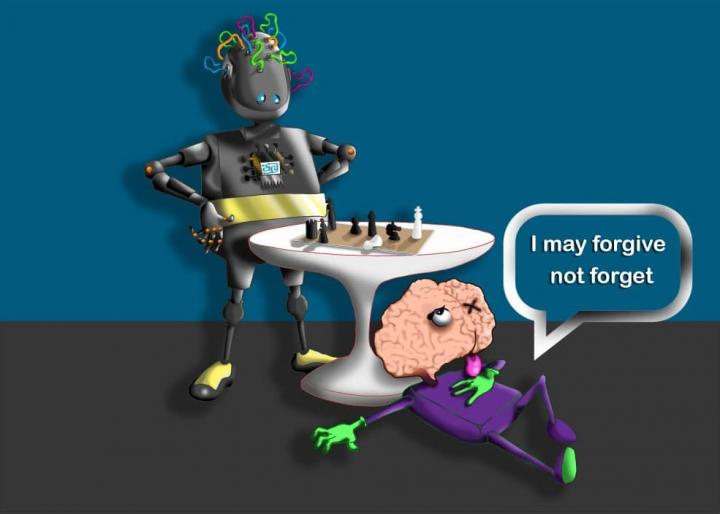
Image: Shahab Mohsenin
The new research shows otherwise: the fewer number of memories stored using the brain strategy depends on such unrealistic assumption. When the simple strategy used by the brain to change the connections is combined with biologically plausible models for single neurons response, that strategy performs as well as, or even better, than AI algorithms. How could this be the case? Paradoxically, the answer is in introducing errors: when a memory is effectively retrieved this can be identical to the original input-to-be-memorized or correlated to it. The brain strategy leads to the retrieval of memories which are not identical to the original input, silencing the activity of those neurons that are only barely active in each pattern. Those silenced neurons, indeed, do not play a crucial role in distinguishing among the different memories stored within a same network. By ignoring them, neural resources can be focused on those neurons that do matter in an input-to-be-memorized and enable a higher capacity.
Overall, this research highlights how biologically plausible self-organized learning procedures can be just as efficient as slow and neurally implausible training algorithms.
Comments