Although I’m a trained and credentialed epidemiologist, and an
ardent supporter of the professional discipline as a foundational science that
underlies legitimate public health efforts, several of my past blogs (Bond
2016 and Bond
2017) have remarked on the many limitations of observational
epidemiology1 research for establishing disease causation.
Of course, I’m not the only such critic writing
about it. Of late, observational studies have been getting a particularly
bad rap in the popular press as too unreliable. This has been especially true
with respect to their application to the topic of nutrition and health, whereby
some scientists have begun to challenge whether any further observational
research can even be justified, expressing their strong preference for instead on
experimental epidemiology in the form of randomized clinical trials
(RCTs) (Ioannidis
2019, Carroll
2019 and Maki
et al 2014).
During a forty plus year career, my personal interests have focused on applying observational epidemiology research to questions of chemical exposures and their relationship to occupational and environmental health. More frequently, that focus has narrowed even further to epidemiology research that purportedly identifies chemicals as endocrine disruptors (EDCs). Just as with nutritional epidemiology, the field of EDC epidemiology is also rife with controversy. Not surprisingly, the challenges inherent in researching dietary factors and chemical exposures are remarkably similar (e.g., long latency periods between the offending exposures and the onset of relatively uncommon diseases; lack of reliable estimates of exposure levels; sorting-out the individual contributions of single factors from highly correlated, mixed exposures; relatively weak associations, etc), so by extension some might legitimately question the value of observational epidemiology to this line of inquiry as well.
Although it is true that RCTs help to avoid most of the problems inherent in observational epidemiology studies, they are not without their own issues, and not all nutrition and health research questions, and certainly not questions of adverse health effects from chemical exposures, can be ethically addressed by randomly allocating humans into exposed and unexposed groups. Thus, even with their many limitations, it will continue to be argued that observational epidemiology studies often provide some of the most relevant scientific evidence available and so they will undoubtedly continue to play a role for the foreseeable future. Underscoring this is a recent announcement that Apple is collaborating with the Harvard School of Public Health to trial the use of some of its smartphone apps to facilitate observational studies of factors influencing women’s health.
And while we can expect observational studies to continue to be done, it is important to note that when it comes to generating quality scientific evidence NOT ALL EPIDEMIOLOGY STUDY DESIGNS ARE CREATED EQUAL! This is a fact that is often not appreciated or acknowledged, even by some who are directly engaged in occupational and environmental health research and policy making. I was reminded of this just in the past few days when reviewing published literature that purports to identify some chemicals as EDCs. The majority of the studies I found on the particular topic were conducted using a cross-sectional design, and the inherent limitations were often either downplayed or even ignored by the authors who went ahead and over-hyped their findings anyway. Moreover, all too often those who conduct and publish systematic reviews of the available epidemiology literature linking a chemical and health effects are guilty of attributing too much weight to cross-sectional studies. For this reason, I thought a blog devoted to the topic of cross-sectional studies would be timely.
As you will learn, cross-sectional studies are among the weakest of observational epidemiology study designs and the results they yield should be accorded far less weight than the results generated from studies employing cohort2 or even case-control3 designs (see the attached Figure for a hierarchy of evidence from epidemiology study design types). Supporting my contention, just recently, the European Food Safety Authority opined that they considered the evidence from cross-sectional studies as too unreliable to give them much weight in their safety assessments.
What is a Cross-Sectional Study?
It is a research tool used to capture information based on data gathered at a specific point in time. The data collected is from a target population with varied characteristics and demographics known as variables. Age, gender, income, education, geographical locations, ethnicity and health status are all examples of variables. The variables, or demographics, used in a single study are based on the type of research being conducted and on what hypothesis the study aims to reject or not.
Cross-sectional designs are NOT exclusive to epidemiology, but are used in many fields, including (but not limited to) business, psychology, social science, retail, education, religion, and government. In each of these fields, cross-sectional research provides data that informs all kinds of actions.
The political polling sponsored by election campaigns or the news media is a prime example of a cross-sectional study design, and the recent notable failures of them to accurately predict outcomes should immediately flash warning signs about their potential limitations.
In epidemiology and public health, cross-sectional methodology is most often used to assess the burden of disease or health needs of a population, and is therefore particularly useful in informing the planning and allocation of health resources.
Types of Cross-Sectional Studies
In epidemiology, there are two types of cross-sectional designs:
·
Descriptive
They may be purely descriptive and used to assess the frequency and
distribution of a particular disease in a defined population. For example, a
random sample of students attending schools across the city of Detroit may be
used to assess the prevalence of asthma among adolescents. Nearly all epidemiologists would argue that
this is the most useful application of the cross-sectional design.
· Analytical
They may be analytical and used to investigate the association between a potential risk factor and a health outcome. However, this type of study is limited in its ability to draw valid conclusions about any association or possible causality because the presence of risk factors and outcomes are measured simultaneously. It is therefore not possible to confidently infer whether the disease or the exposure came first, so causation can only be inferred by additional studies employing more rigorous designs. The collection of information about risk factors is also often retrospective, which increases the risk that technical bias, caused by differential faulty recall among those with and without the health outcome under study, will skew the results.
In practice cross-sectional studies often include an element of both descriptive and analytical approaches.
Strengths of Cross Sectional Studies
· They are relatively quick, cheap and easy to conduct (no long periods of follow-up).
· Data on all variables is only collected once which simplifies things.
· Able to measure prevalence for all factors (health outcomes and potential risk factors) under investigation.
· Multiple outcomes and exposures can be studied.
· The prevalence of disease or other health-related characteristics are important in public health for assessing the burden of disease in a specified population and in planning and allocating health resources.
· Good for descriptive analyses and for generating hypotheses.
Weaknesses of Cross-Sectional Studies
· Difficult to determine whether the exposure or outcome came first (there may be a phenomenon referred to as “reverse causality” whereby instead of the risk factor under investigation actually being a cause of the health outcome, the health outcome itself directly influences measurement of the risk factor)
· Incapable of rigorously testing hypotheses
· Not suitable for studying rare diseases or diseases with a short duration
· As cross-sectional studies measure prevalent rather than incident cases, for life-threatening diseases the data will always reflect determinants of survival as well as etiology
· Associations identified are difficult to interpret
· Susceptible to a number of technical biases that can skew the results
Example of a Cross-Sectional Epidemiology Study
A national example of a cross-sectional study is the annual National Health and Nutrition Examination Survey (NHANES) which is a program of studies, begun in the early 1960’s, designed to assess the health and nutritional status of adults and children in the United States. The survey examines a nationally representative sample of about 5,000 persons located across the country each year. The NHANES interview includes demographic, socioeconomic, dietary, and health-related questions. A health examination component consists of medical, dental, and physiological measurements, as well as laboratory tests administered by highly trained medical personnel. Included is the measurement of trace levels of about 300 chemicals in blood and urine samples obtained from NHANES participants, called biomonitoring. Findings from the NHANES survey are used to determine the prevalence of major diseases and risk factors for diseases. Data from this survey are also then freely available to academic scientists to conduct subsequent research, including studies that try to draw statistical correlations between low level chemical biomonitoring measurement results and a host of health outcomes.
Issues That Must be Considered in the Design of Cross-Sectional Studies
· Choosing a representative sample
A cross-sectional study should be representative of the target population in order to draw valid inferences from the sample of persons studied to that larger population. Participants should be randomly selected. Attempts should be made to include everyone in the random sample so as to avoid what is referred to as selection bias which can skew the results.
· Sample size
The sample size should be sufficiently large to estimate the prevalence of the health conditions of interest with adequate precision. The larger the study, the less likely the results will be due to chance alone. It is too often the case that the investigators compromise on sample size because of cost and practical considerations and thus chance remains a likely alternative explanation for the results.
· Data collection
Measurement of exposures and health outcomes should be done with the most accurate methods available. However, again it is too often the case that investigators compromise because of cost and practical considerations and employ less than ideal measures and this greatly limits the validity of the results. As an example, a single point in time blood or urine sample is too frequently relied on to estimate life-time exposure to a chemical that is already known to be quickly metabolized and eliminated from the body and whose values can fluctuate tremendously throughout a 24 hour period within a single individual. The validity of the results of such studies is highly suspect. Or, information on exposures, potential confounders and health outcomes are obtained by questionnaire rather than direct measurements, again leading to strong doubts about the validity of the findings.
· Collinearity of Putative Risk Factors
The highly correlated nature of some putative risk factors adds complexity to the interpretation of results from cross-sectional studies. For example, Dr. Leonardo Trasande and his colleagues published a recent study which tried to link various bisphenols detected in urine and the prevalence of obesity and noted that their analysis was “ …further complicated because food and beverage packaging, in particular, the lining of aluminum cans, contains bisphenols. Therefore, those who consume more of these products are more likely to have higher exposure levels and, perhaps, are more likely to be obese.” In such situations, statistical modeling may not be able to sufficiently control for and eliminate potential confounding by such highly correlated factors and can yield misleading results.
· Potential bias in cross-sectional studies
Non-response is a particular problem affecting cross-sectional studies and can result in bias of the measures of health outcomes. This is a particular problem when the characteristics of non-responders differ from responders. Recall bias can occur if the study asks participants about past exposures, because it has been repeatedly demonstrated that those who are afflicted with a medical condition tend to scour their memories and even exaggerate past exposures more so than those who are otherwise healthy creating false-positive associations.
· Analysis and interpretation of cross-sectional studies
All factors (exposure, outcome, and confounders) are measured simultaneously, and thus only prevalence rates (rather than incidence rates) can be estimated. As discussed earlier, it is therefore not possible to confidently infer whether the disease or the exposure came first, so causation can only be inferred or refuted by additional studies employing more rigorous study designs.
Implications of the Limitations of Cross-Sectional Studies
Given the substantial limitations of cross-sectional studies noted above, there are several important implications for research on chemicals purported as EDCs that must be considered going forward.
· Just because cross-sectional studies are quick, cheap and easy to conduct, it certainly does NOT justify their conduct or publication in the literature. This is especially the case when some of the acute problems discussed above, such as poor quality exposure estimates and high collinearity with other more established risk factors, are likely to undermine the validity of the results. I sometimes wonder if the sole motivation for undertaking many of these studies is simply to pad the authors’ curriculum vitae for the purposes of career advancement rather than the supposedly altruistic purposes they trumpet.
· One problem with epidemiology is that there are plenty of “environmental health scientists” who lack formal and rigorous epidemiology training and yet undertake such studies leading to poor quality execution, results and over-hyped interpretation. Unlike animal toxicology studies, which tend to be capital intensive, the barriers to conducting observational epidemiology studies more often relate to labor costs, but then again graduate students work cheap.
· Because cross-sectional studies can only suggest and NOT actually test causal hypotheses, it is difficult to justify their repeated conduct on the same topic after an initial study demonstrates a putative association. Multiple cross-sectional studies, especially when they are of near identical design conducted by the same research group, do not materially add to the weight of evidence for inferring a causal relationship. Rather than conduct yet another cross-sectional study to only confirm the first one, it’s definitely time to move on and conduct a more rigorous prospective cohort study.
· Finally, given the many limitations inherent in cross-sectional studies, those who prepare systematic literature reviews and/or meta-analyses should clearly weight them less and separate them out from case-control and cohort study results when presenting summaries of the relations between exposures and disease outcomes.
Thus, in conclusion, it is wise to practice caveat emptor when encountering results generated from cross-sectional epidemiology studies.
Some Suggested Further Reading
· Carlson MDA, Morrison RS. Study Design, Precision, and Validity in Observational Studies. J Palliat Med. 2009 Jan;12(1):77-82. https://www.ncbi.nlm.nih.gov/pmc/articles/PMC2920077/
· Gordis L. Epidemiology. 4th ed. Philadelphia: Saunders Elsevier; 2008.
· Levin KA. Study design III: Cross-sectional studies. Evid Based Dent. 2006;7(1):24-5. https://www.nature.com/articles/6400375
ENDNOTES:
1Observational epidemiology studies are distinguished by the fact that the investigator does not manipulate the conditions of exposure for the subjects under study in any way, but instead merely observes what has often been described as the “natural experiment”. Chance, bias, and confounding are more likely to occur and must always be considered as possible explanations for an observed association between an exposure and an outcome.
2In a cohort study, the groups of persons to be studied are defined in terms of characteristics manifest prior to the onset of the disease(s) under investigation and are observed over a period of time (into the future for prospective cohort studies or from some point in the past to the present for retrospective cohort studies) to determine the frequency of disease among them.
3In a case-control study, the groups of persons to be studied are selected on the basis of whether they do (cases) or do not (controls) have the disease under investigation, and the groups are then compared with respect to existing or past characteristics judged to be relevant to the causes of the disease.
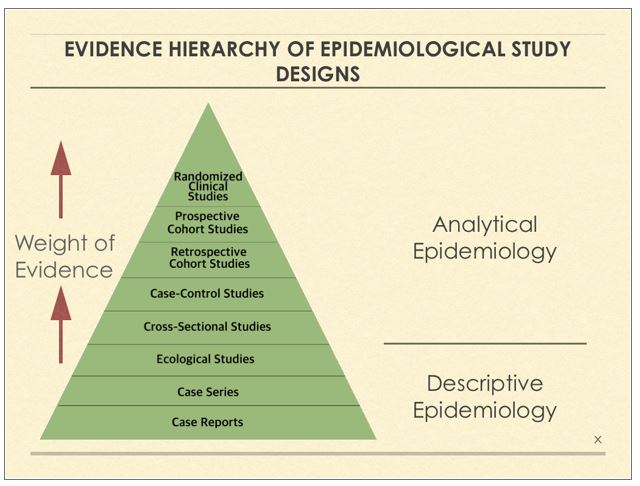
Comments